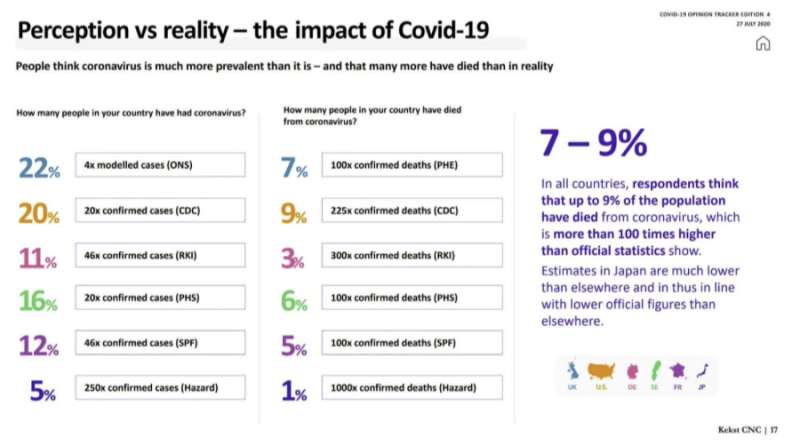
Take a close look at the above slide from an international poll conducted a few months after Covid struck: This is what effective propaganda looks like. And the true effect was even greater, because the ‘real world’ numbers used to calculate how badly people exaggerated the risks of Covid were of course themselves derived from. . . the world’s preeminent propaganda organizations (masquerading as public health agencies). Who were themselves already wildly exaggerating the risks of Covid.
The art of effective propaganda is an encompassing discipline that requires careful and thorough study — and review — from time to time. For the beginner, it can be very difficult to master. Even the experienced propagandist can at times fall into the trap of thinking that creating and disseminating propaganda is a straightforward enterprise – which is a good way to win a permanent all-expenses paid Siberian vacation. It is not usually so simple a task to befuddle the entire society every day, 365 days a year, indefinitely.
The following short guidebook will provide the aspiring propagandist, WEF lackey, Communist Apparatchik, Woke Marxist, and seasoned government bureaucrat alike with the tools and knowledge necessary to develop their promising talent into full-bloom mastery of the art of propaganda.
This book is a bit long!! So don’t feel as though you must read it start to finish in one shot, for that is a recipe to burnout and to not retain the critical information contained within.
This manual is divided into the following sections:
Section I. Definitions – How to redefine words, terms, and metrics to keep them in line with the regime narrative
Section II. Curating Data – How to hijack the processes of recording, reporting, and publishing data
Section III. Vetting which data are considered to be part of Official Science – How to vet and data and dispose of regime nonconforming data so it never appears in any Official Science or regime datasets
Section IV. How to rig a study – Exactly what it sounds like
Section V. Doctoring the datasets – Sometimes, you will need to go in and do a little data ‘surgery’ to modify the content of databases that contradict regime talking points you can’t simply eradicate
Section VI. Control the standards of evidence – How to set up a hierarchy of evidence that puts regime-friendly Science at the top and regime-unfriendly science at the bottom (of the Mariana Trench)
Section VII. The Ecclesiastical Authorities of Science – How to ensure that Scientific Authorities reliably parrot the regime’s facts and narratives
Afterword – Tying it all up nicely, like one of Peter Hotez’s bow ties (he’s a particularly grating Regime Celebrity Scientist)
Section I – Definitions
“He who controls the language controls the masses.”
— Saul Alinsky, Rules for Radicals
How we define concepts or categories determines what tidbit of the real world they communicate or represent – or what they don’t communicate or represent.
Malleable definitions, and an arbitrary and capricious standard for assigning definitions, are an absolute must for any effective propagandist. Despite the best efforts, even seasoned, expert propagandists will inevitably confront situations where the curated data that exists, or people’s lived experience, are problematic to the official regime narrative.
Effective propaganda therefore requires the capacity for nimble and highly adaptive flexibility to control the content of data, especially pre-existing conventional metrics that the public is accustomed to hearing about which are notoriously difficult to simply make disappear (unlike the ease by which you can vanish a dissident scientist off of YouTube or Facebook). For example, you won’t be able to avoid talking about “deaths” in the context of a novel Dreaded Disease Pandemic – the primary way people will relate to gauging the severity of a disease will always be first and foremost “How many people died from the disease?” But you can change what “death” refers to in the context of the novel Dreaded Disease if you want to increase or decrease people’s sense of how deadly it is.
In practice, this means that when the normal understanding of a term or concept shows that the reality does not quite fit the regime’s desired narrative, just change a few definitions and voilà, problem solved.
As many a prominent communist propagandist throughout history has also observed, “He who controls the language rules the world.”
There are a variety of ways to alter or transition definitions from problematic to acceptable:
I-1. Limit a Definition
If the conventional definition of something includes concepts, data, or information that is at odds with regime dogma, limit the definition so it no longer includes the unwanted information. There are plenty of ways to do this. So we’ll list a few of the more common types of characteristics you can use to effectively limit a definition: Limit the definition by time interval: Suppose that vaccinated people get the Dreaded Disease at very high rates in the first 30 days following vaccination, and after 90+ days from vaccination, with the Glorious Vaccine. This is a big problem, because people will think that the Glorious Vaccine is not effective:
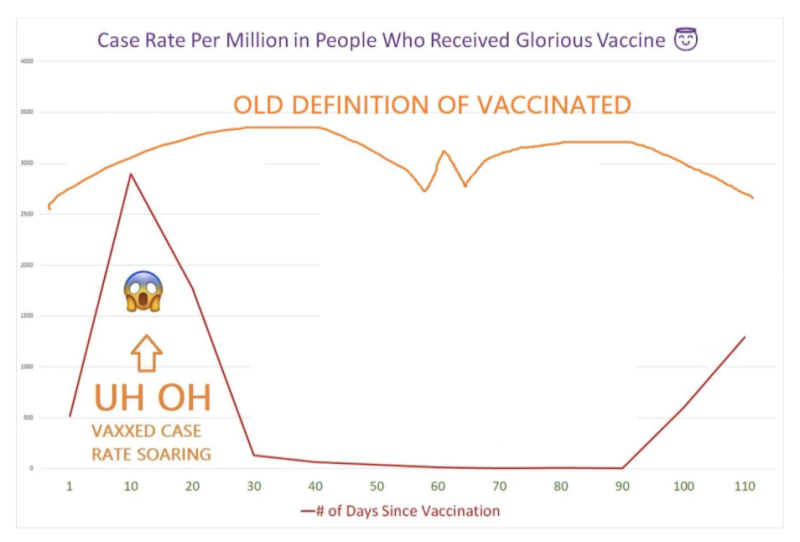
In plain English, what you see on the above chart is that the number of cases per million people goes as follows:
- Before vaccination: 500 cases of Dreaded Disease/million people
- 10 days after vaccination: 3,000 cases of Dreaded Disease/million people
- 20 days after vaccination: 1,700 cases of Dreaded Disease/million people
- 30 days after vaccination: 100 cases per million people
That’s very inglorious efficacy for the Glorious Vaccine – something that cannot be allowed to stand. One solution is to simply change the definition of ‘vaccinated’ to mean someone who is between 30 and 90 days after being injected with the Glorious Vaccine – in other words, anyone who is within 30 days of being vaccinated, or after 90 days from vaccination, is not considered ‘vaccinated:’
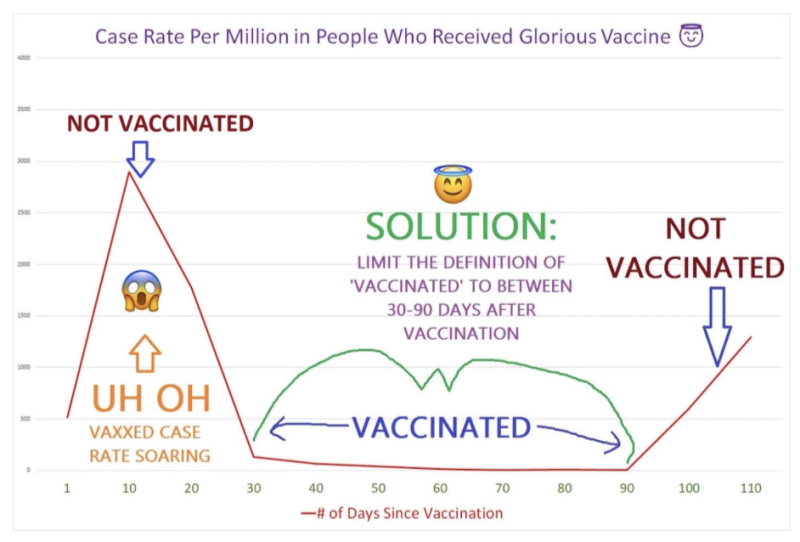
This particular tactic was pioneered by pretty much every public health agency in the civilized world, where the definition of ‘fully vaccinated’ for the Covid vaccines was limited to “14 days after your second dose:”
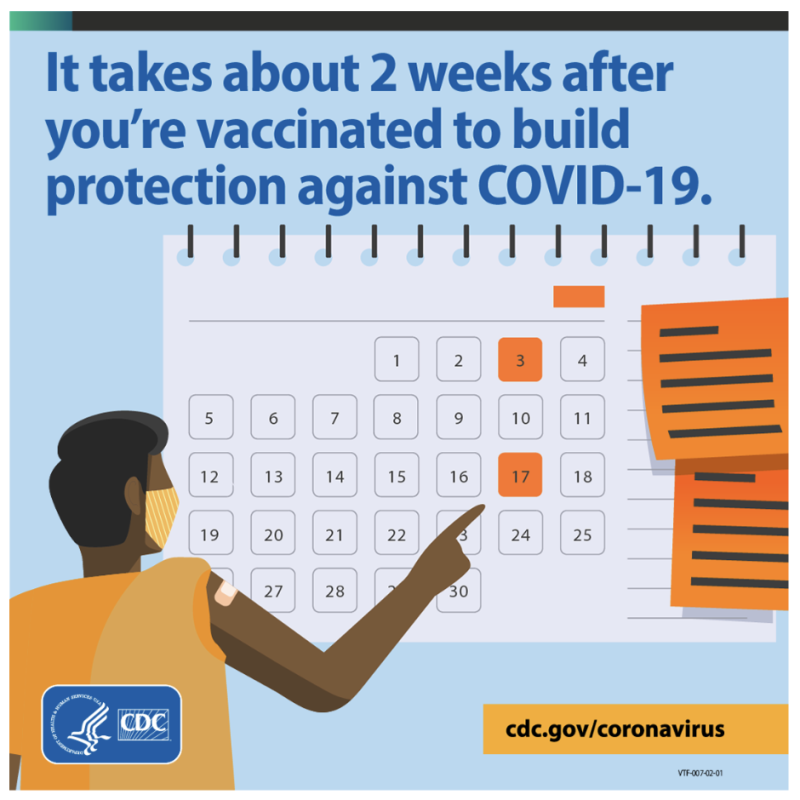
Limit the definition by quantity, such as the number of exposures – for instance, if a bunch of people who received 1 dose or 5 doses of the Miraculous Treatment Mirafaucivir died (the first dose kills off people who are particularly susceptible to its toxicity, and 5 doses is too toxic for pretty much anyone), limit the definition of ‘treated with MiraFaucivir’ to between 2-4 doses:
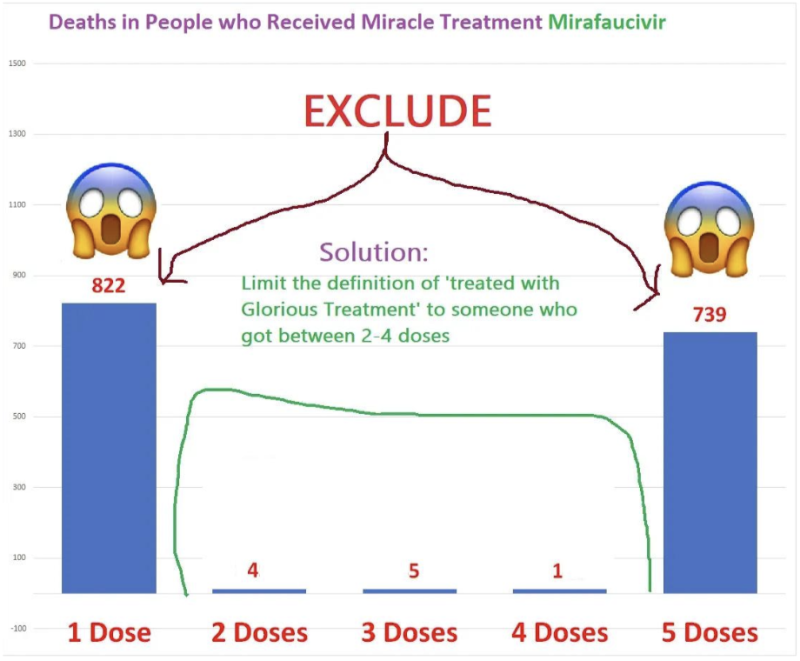
Limit a definition by adding absurd conditions into the definition that are almost impossible to fulfill. For instance, you might try using the following conditions to limit the definition of a ‘vaccine death’ in the context of a mass vaccination campaign with the newly-minted Glorious Vaccine:
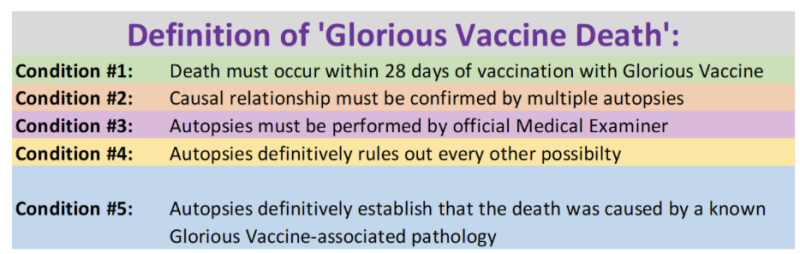
It’s pretty hard to ever manage to get a ‘confirmed’ case of someone dying from the Glorious Vaccine under conditions like these.
(You must remember to obstruct autopsies as much as possible to make this example definition fully effective.)
I-2. Expand a Definition
Conversely, sometimes you may want more of something than there actually is. Expanding definitions is a great solution – just reverse the above instructions for limiting definitions.
So if you need more deaths from the Dreaded Disease than there are people actually killed by the Dreaded Disease, you can expand the definition of a ‘Dreaded Disease Death’ to ‘any death within 30 days of a positive test,’ and just like magic you have a full-scale pandemic on your hands.
To illustrate this, suppose that after 12 months of Dreaded Disease circulation, only 7 people per 100,000 infections were actually killed by the Dreaded Disease – not exactly scary. You pull a little switcharoo and expand the definition of a ‘Dreaded Disease Death’ to something like what the CDC pulled – “any death within 30 days of testing positive for the Dreaded Disease.” Since plenty of people die every day, if you mass test them all, you will inevitably ‘discover’ a whole boatload of dead people who happened to have the Dreaded Disease when they died, even though they were killed by something completely unrelated like cancer or a car crash. Look at what a difference this makes:
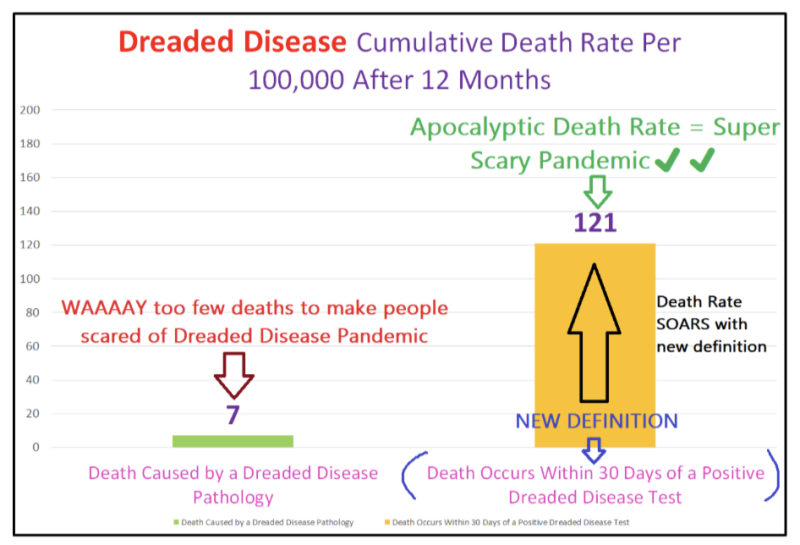
New York State offers a classic illustration of how to expand the definition of “Dreaded Disease Death” to create the appearance of a once-in-history super-duper scary apocalyptic pandemic – just look at the following gorgeous open-ended definition for a ‘probable’ Covid death:
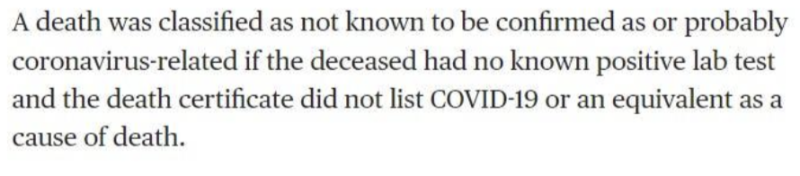
NOTE OF CAUTION: You must always take care to NEVER, EVER, EVER – EVER!!! – articulate to the public how you’re gaslighting them in clear, concise language they can understand. The following unforced error in 2020 from Illinois Public Health Director Dr. Ngozi Ezike is the sort of thing that gets you a quick one-way ticket to the Gulag – she actually said the following at a public press conference (see embedded video below):
“So, the case definition is very simplistic. It means at the time of death, it was a Covid-positive diagnosis. So that means that if you were in hospice and had already been given a few weeks to live and then you also were found to have Covid, that would be counted as a Covid death. It means that, technically even if you died of a clear alternate cause, but you had Covid at the same time, it’s still listed as a Covid death.”
She was doing the right thing of course by using such a wonderfully expansive definition for Covid deaths, but she stupidly and carelessly let the cat out of the bag for the whole world to see. That’s the kind of careless blunder that can demolish an entire propaganda campaign overnight. And also the kind of thing that can be a career-ender (or worse):
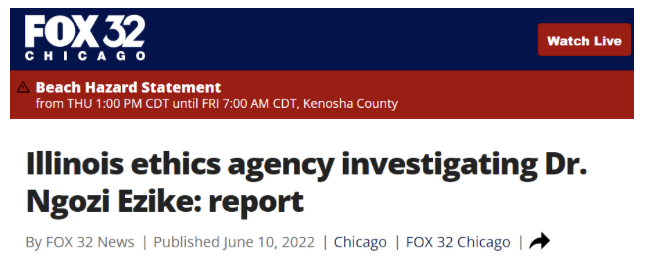
I-3. Invent a Brand New Definition
Sometimes it is simply not possible to hide the common understanding of something by merely playing with the definition at the margins. In that case, you can take the gutsy step of redefining a word, concept, or category altogether to fit your propaganda needs. Just beware that it may be a tad more difficult to convince people that the old definition is a figment of their imaginations.
Take the CDC (yup, we’re gonna be quoting CDC a lot; they are the preeminent health propaganda organization in the world after all), which changed the definition of ‘vaccination’ multiple times over a span of 6 years:
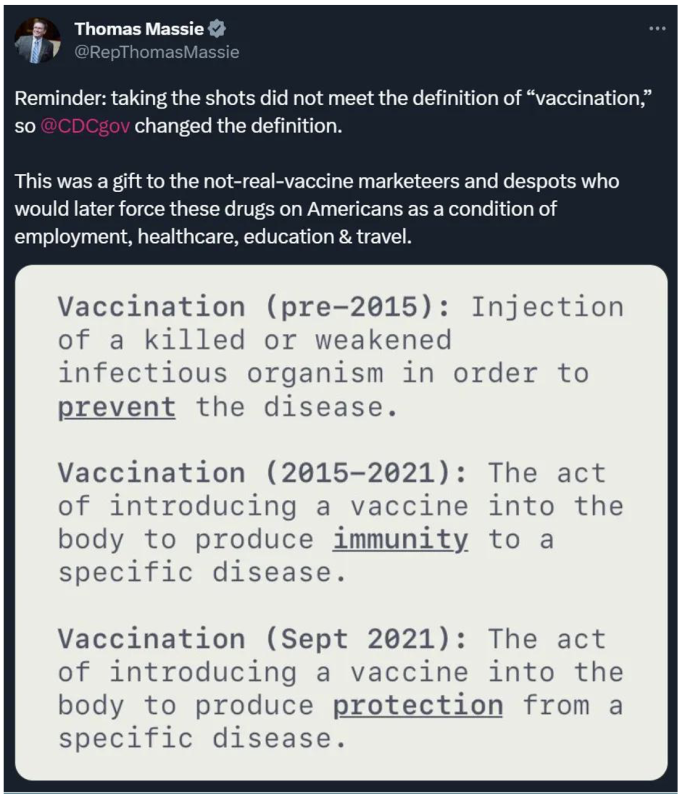
Sidebar: The above tweet offers a lesson in the need to control rogue legislators who might try to dissent or even expose your propaganda efforts. You don’t need the additional headache of dealing with clear evidence of your linguistic treachery broadcast to the public from the floor of Congress or Parliament (or the even bigger headache of being banished to Siberia as the fall guy for allowing such a thing to happen).
On occasion, you may even find that you are trapped by the ordinary conversational meaning of words, where they highlight something you can’t afford people paying attention to. Should this occur, you will be forced to implement a fundamental change to the very essence of the language. This is a sort of nuclear option for when you can’t hide something any other way, and also can’t afford to not hide it. (Beware!! Such an audacious endeavor comes with a significant degree of difficulty as many people will be inclined to resist such open and bold language transitioning – similar to how many unenlightened Luddites resist going along with gender transitions).
For example, take the term “peaceful protest:”
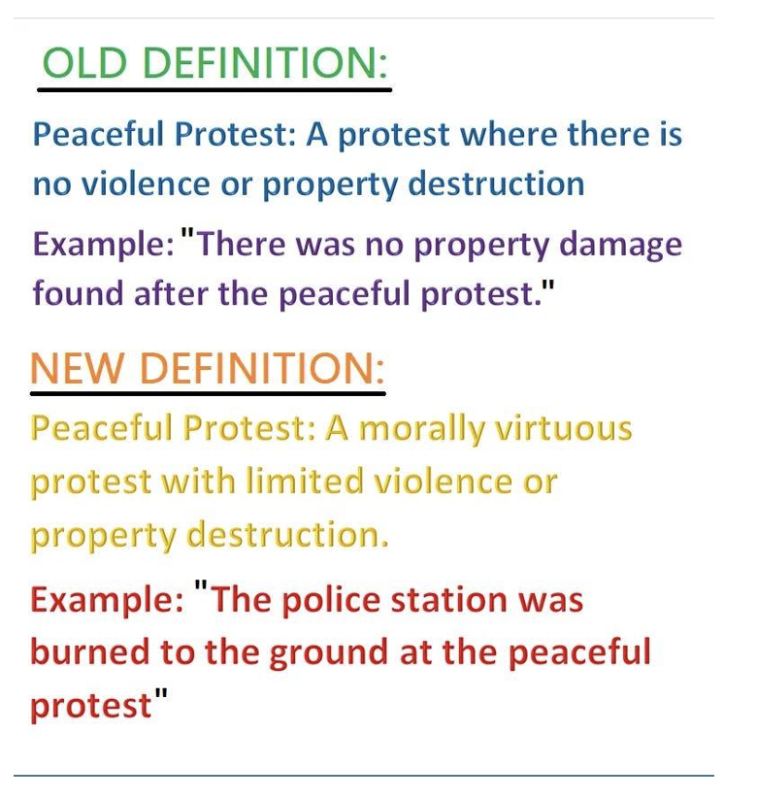
Of course, ‘limited’ is a subjective term whose precise contours are ill-defined, which gives you a lot of latitude to apply the description to almost anything regardless of how incoherent or misfit the application is, as evidenced by this real-life media report that needs no further description:
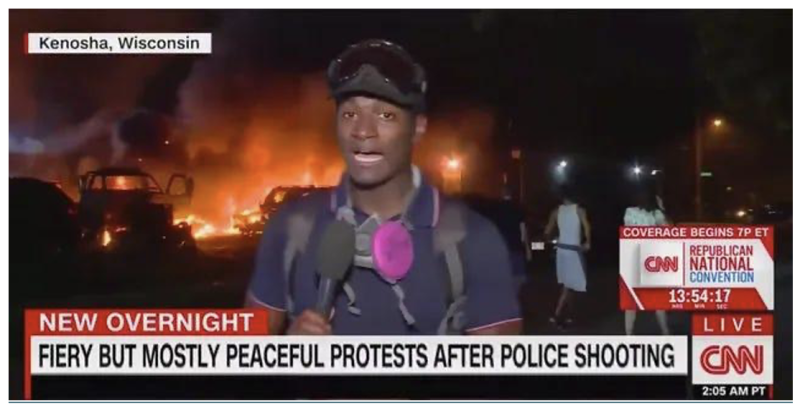
I-4. Combine Categories
Sometimes, it just isn’t practical or feasible to mold the data simply by changing definitions. Not to worry though – if you can’t change the definition, you can instead change the datapoint or category itself that folks are used to the word or phrase referring to. People aren’t attuned to subtle or nuanced differences in categories or data points, and the media helpfully conflate most things anyway, making this an easy and convenient trick. For instance, you can try:
- Combining different age groups:
Suppose the Glorious Vaccine is causing a bunch of kiddies to turn into zombies. That’s pretty bad for the regime. (Which means you should reassign a few scientists to work at a climate research station in Antarctica for the remainder of their careers. Without socks.)
First, you must always refer to this novel condition as “Safe and Effective Transformation into Carnivorous Zombie.” The reason for the carnivorous part is simple: ‘flesh eating zombie’ sounds too scary, and plain ‘zombie’ feels like the zombies are basically dead – i.e. the precious kiddies are dead – neither of which is an impression you want people to come away with. (Even though our hypothetical example here is unlikely to materialize in practice, the principle is relevant and applicable to any situation: you must always name something in a way that conveys a sense of what you want people’s impressions to be.)
Second, because the rate of Zombification in the 12-17 age cohort is so high that it is obvious to anyone who looks at the data (below chart), you will probably have to deal with that. So instead of presenting the data broken down by age, where people will immediately notice the surge of kiddie zombification, present the data as a combined age group that is big enough to hide or launder out the signal:
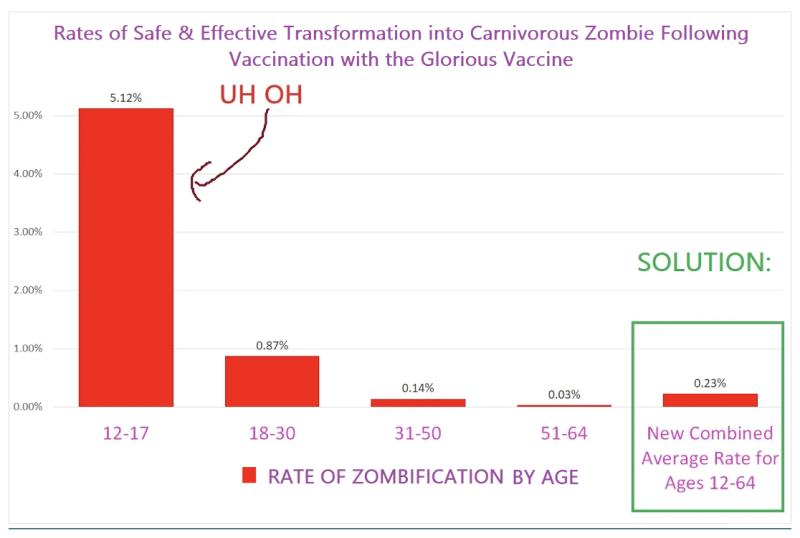
Now nobody will notice that the data shows a clear risk to kiddies of being turned into carnivorous zombies by the Glorious Vaccine.
Or conversely, supposing that the youngsters aren’t dying from the Dreaded Disease at high enough rates to scare the mommies, you can present Dreaded Disease death data from a combined age group of 0-50 that makes it seem like there are soooo many deaths from a group that includes the kiddies:
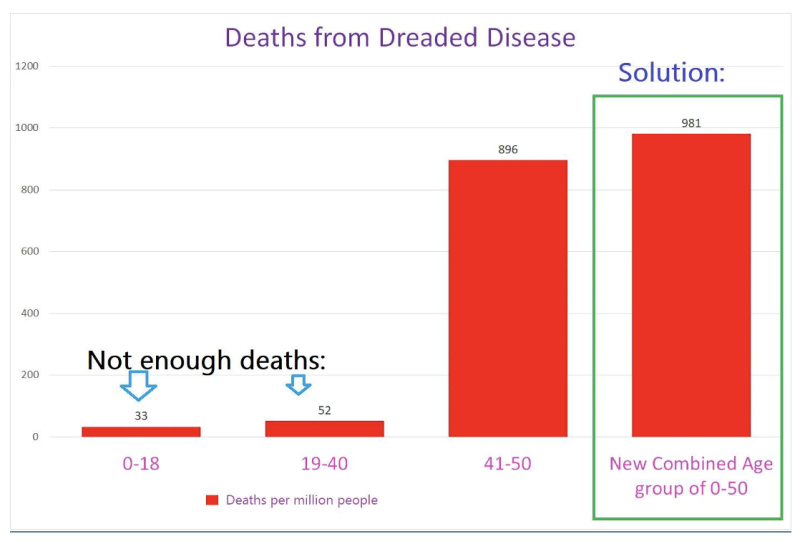
- Combining different demographic cohorts:
Same idea as the age groups; suppose you need to avoid the citizens figuring out that the Dreaded Disease is really only dangerous to the morbidly obese people – which is bad:
- firstly because then they won’t be scared of the Dreaded Disease
- secondly because people might start to question whether fat is healthy, which you can’t allow because they might begin to question the regime narrative regarding ‘fat positivity’ and then who knows what else afterwards.
So you should just present the Dreaded Disease death data using a combined category that covers all types of weight identities:
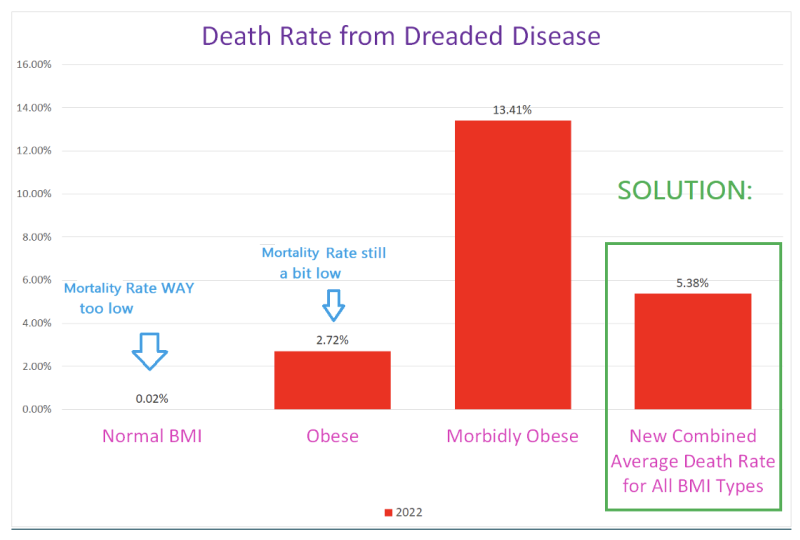
- Combining different time periods
Suppose you notice that the deaths from the Dreaded Disease are decreasing month over month – which can be catastrophic to regime plans that require the people to believe that the Dreaded Disease Pandemic is in full circulation for another few months. If the people get the idea that the Dreaded Disease is winding down, well, that’s a lot of lost opportunity to use the Dreaded Disease crisis as a means of effecting societal transformation to consolidate and solidify the regime’s power.
So instead of presenting the death data by month, combine all three months into a new category of “monthly average over the three months” which will mask the decrease from January to March, illustrated below:
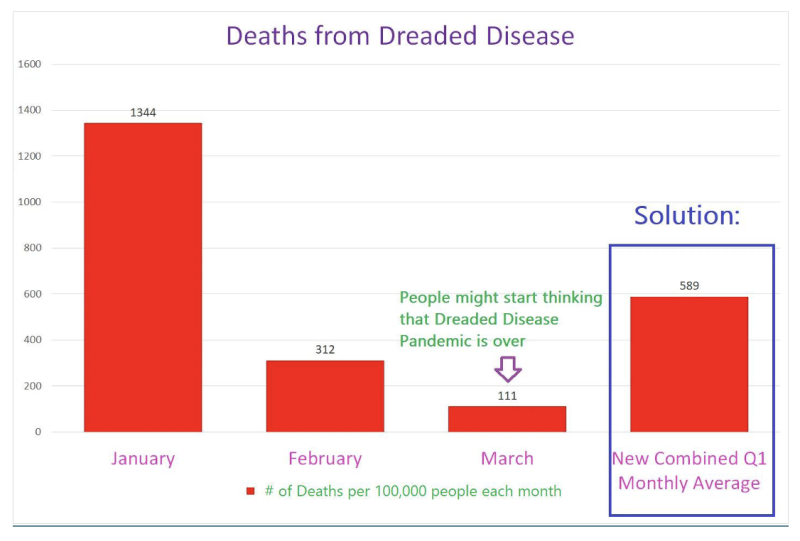
- Combining different geographical jurisdictions
Suppose that there’s a rogue state within the country that is making problems for the regime that doesn’t follow regime guidance for dealing with the Dreaded Disease, which we’ll call Death Santistan. If they show better or even equal results to the rest of the country where they are good citizens and follow regime guidance, that would be pretty bad. Suppose further that there’s a city or county within this bad state that is a loyal regime county following all regime guidance but whose death rate is much higher than the rest of Death Santistan. Which is very very bad. Solution? You can present data from the entire state so people can’t tell that the loyal county following regime guidance has a death rate 10 times the rest of the state. There’s even a bonus benefit: you can point to the whole state of Death Santistan as a failure because the loyal regime county will make the whole state look much worse!!
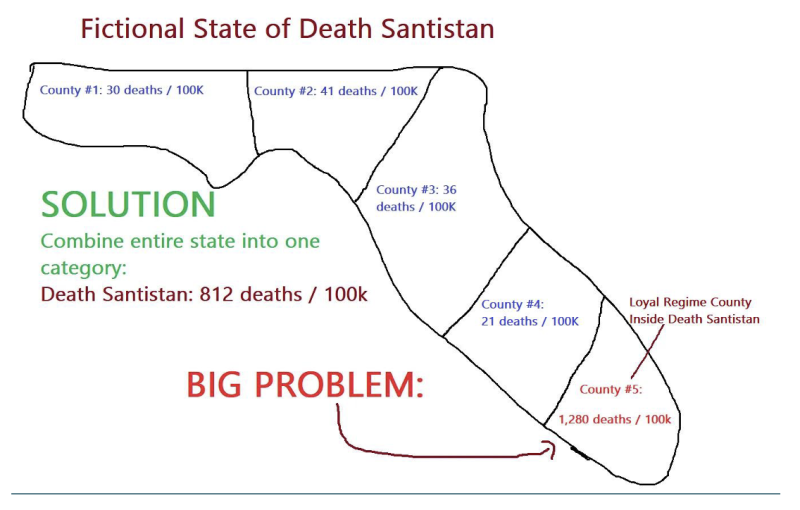
Combining all the cities and counties in a disloyal state to hide the problems unique to loyal regime cities is one of the go-to propaganda tactics used to try and hide unflattering information such as the vastly higher crime rates in regime-loyal cities compared to cities controlled by the evil opposition.
(Sidebar: High crime rates are a good thing of course that is a deliberate choice of the regime by design – high crime rates are useful for the regime because instability makes people more willing to accept tyrannical government as a solution.)
To illustrate, here is a brilliant piece of gaslighting from one of the mainstay regime media mouthpieces:
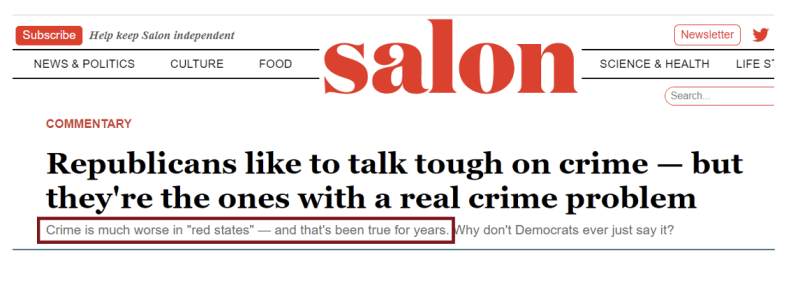
Look at the subtitle in the crimson box – see how they adroitly finger the red states for the high crime rates that are all in the blue cities within the red states but not in the rest of the state where the governance is “red?” Exactly.
- Combining different types of the effect or phenomenon. For instance, if there is an increase in a specific subtype of disease condition – like alarming increases in rare cancers following the rollout of the Glorious Vaccine, which might make people question the official regime narrative that the Glorious Vaccine is the safest entity ever created or discovered in universal history – you can use the general category of cancer – which is 1,000x as big – to hide the signal.
Another way to think of combining categories is that you never give out the specific data for different groups or subsets, something that was pulled off to absolute perfection when Covid struck. Consider the following polling results, showing the share of Covid deaths for each age group side by side with the percentage of each age group who were worried they would be killed by Covid. (The blue bars show the percentage of each age group who were worried about getting killed by Covid, the green bars show the percentage of the total number of Covid deaths that were in each age group.)
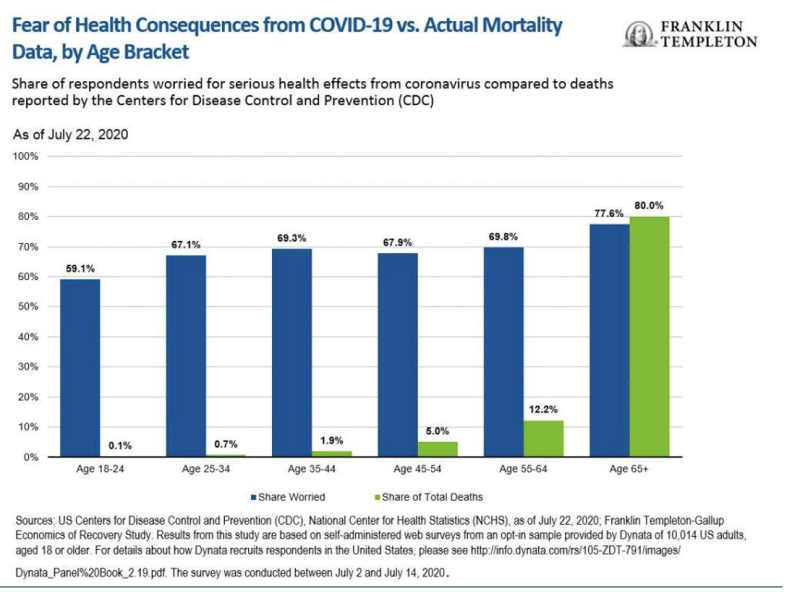
Had people understood what their actual risk of dying was, the blue bars should be at least in the ballpark of the green bars. When the blue bars are dramatically higher, that is the result of brutally effective propaganda by combining all age groups into one category without ever differentiating:
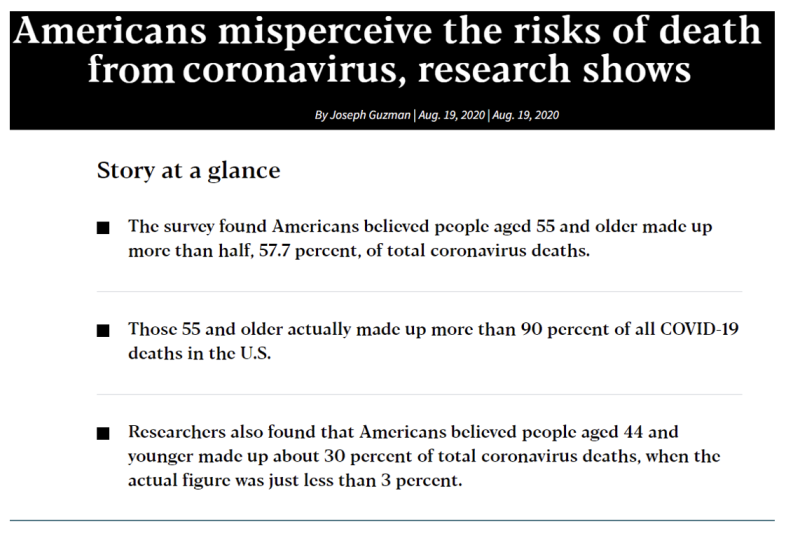
Smashing success indeed!!
I-5. Split Categories
Sometimes you will need to split up a category instead of combining it with another one. Just reverse the framework laid out above for combining categories.
This neat little maneuver is especially useful when you need to get something below the threshold for statistical significance.
Since statistical significance is a pretty important concept in data and Science, it’s a good idea to explain how this works.
Statistical significance as used in conventional medical academic/scientific language basically means that the likelihood of something not due to random chance is less than 5%.
If you flip a coin 10 times, the odds of getting 7 heads because of random chance is 11.72% – NOT statistically significant. If you flip a coin 100 times, the odds of getting 70 heads because of random chance is a miniscule 0.0023% – VERY statistically significant (cuz that’s much less than 5%) – meaning that it is not reasonably attributable to random chance, rather something specific (like cheating) caused the coin to flip 70% for heads.
Why is this? To get 7/10, all you need is two extra coin flips to go your way – going on a bit of a streak. Small deviations like this can easily happen at random. However, to get 70/100 requires 20 extra coin flips to go your way – the odds of getting *20* extra coin flips out of a total of only 100 by random chance are negligible. So if we see 70 heads out of 100 flips, we can presume that there is some kind of cheating going on, because that’s very very unlikely to happen by random chance.
You can use this to your advantage to divide and conquer a statistically significant signal – you can divide a category where there is a statistically significant signal for something against regime doctrine into smaller categories in order to break up the signal from a ‘70/100’ into a bunch of ‘7/10’s that are individually not statistically significant.
So if for example there is a signal that there are more deaths per 100K per year after the Wondrous Glorious Vaccine Campaign, you can publish the death data broken down by age group where no one age group will show a statistically significant increase in deaths (and you can claim that it’s probably leftover excess death from ‘Long Dreaded Disease’ from complications of getting the Dreaded Disease):
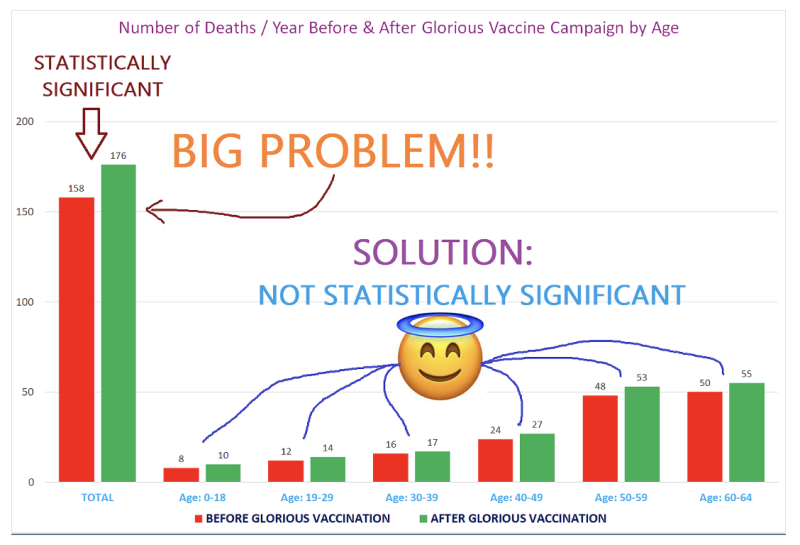
Note of caution: This particular tactic should ideally be combined with something else; otherwise people could reverse-engineer the breakdown by doing a bit of simple arithmetic to add all the age groups together. So make sure to add in other confounding tricks.
I-6. Redistribute / Redraw Categories
A more finely tuned alternative to combining categories outright is to redistribute them – to redraw the lines so to speak. This can be done using any characteristic by which categories are differentiated.
To illustrate, returning to our example of the evil disloyal state of Death Santistan, instead of combining the entire state into one statewide statistic, you can surreptitiously redraw the geographic boundaries of the counties inside the state for the purposes of Dreaded Disease data like this – look at what happens when we change the county borders to the green lines:
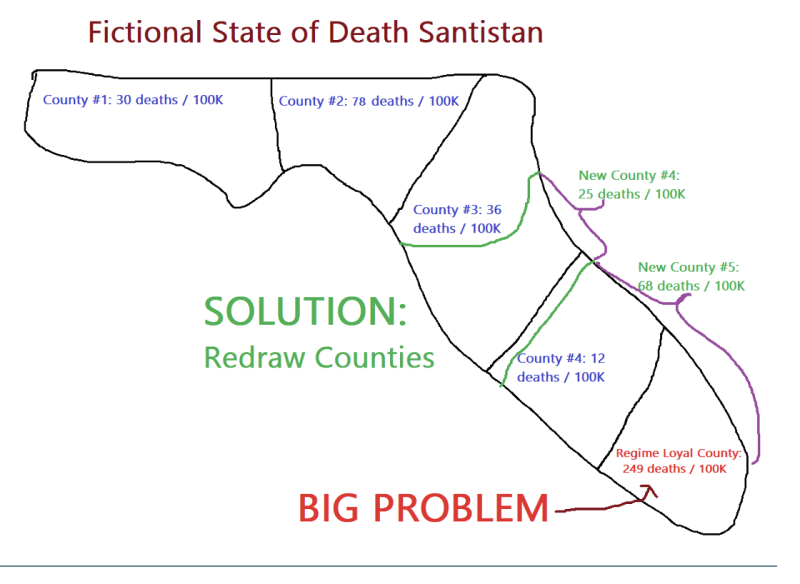
Note: This does not mean you have to literally redraw the counties for political and other purposes like voting districts; all you’re doing is using different borders for the sole purpose of Dreaded Disease statistics. (The population however will assume that you mean the actual counties that exist and will therefore not realize you pulled a fast one over them. It’s called propaganda for a reason.)
I-7. Fluid Definitions
There are times when you may have the paradoxical need to use a specific definition for one thing but must also avoid that specific definition for something else. For such cases, you must act like a dictionary – dictionaries typically have multiple distinct definitions for one word, you can do the same.
For instance, the word ‘woman’ is sometimes defined as ‘an adult human that possesses female anatomical and genetic characteristics,’ such as when discussing a woman’s right to choose; and is sometimes defined as ‘a person who identifies as woman,’ such as in the context of organized sports.
Section II – Curating Data
Even better than plying malleable definitions is avoiding situations that require definition shifting in the first place.
The best way to head off such issues is to curate the data in a way that avoids creating potential headaches, by employing one or more of the following tried and tested methods for corruptly hijacking the curation, organization, and presentation of data.
II-1. Don’t Diagnose or Identify Something
If a patient comes in suffering from multiple neurological deficits after taking the Glorious Vaccine and gets sent home with a Xanax prescription for his “anxiety,” it won’t generate a diagnosis for a neurological deficit in the first place in any database. No diagnosis of a condition that could’ve been caused by the Glorious Vaccine – or diagnostic code in some big government or insurance database – means you will have to employ definitional sleight-of-hand to cover up the existence of diagnosed injuries associated with the Glorious Vaccine. Thus you should ensure the people responsible for diagnosing or identifying problematic or contradictory data/observations to the Perfectly Safe and Effective Glorious Vaccine will avoid doing so.
It is worth emphasizing here that patients are easily gaslit by their own physicians that ‘It’s all in their head,’ even while they know they have serious, life-altering medical injuries that leave them disabled and completely unable to function that they experience daily.
Let’s illustrate this with the following hypothetical scenario:
Regime officials see that in the government-controlled PROPAGANDA Safety Surveillance Database set up to monitor Glorious Vaccine safety –
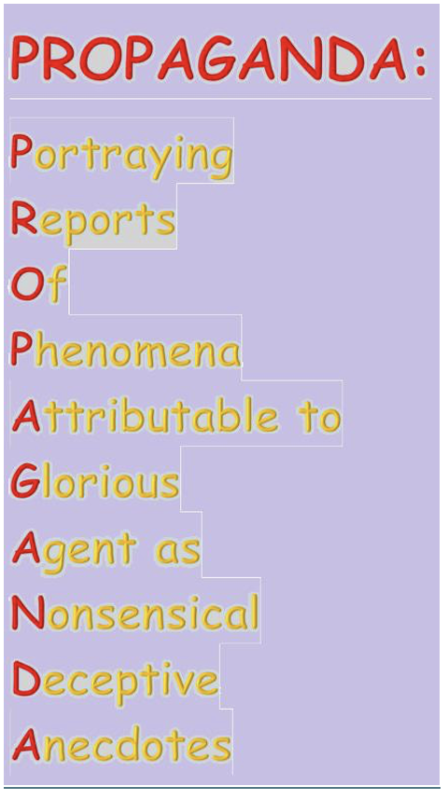
– there is a signal for VAMP Syndrome (Vaccine Associated Metamorphological Phenomena) conditions:
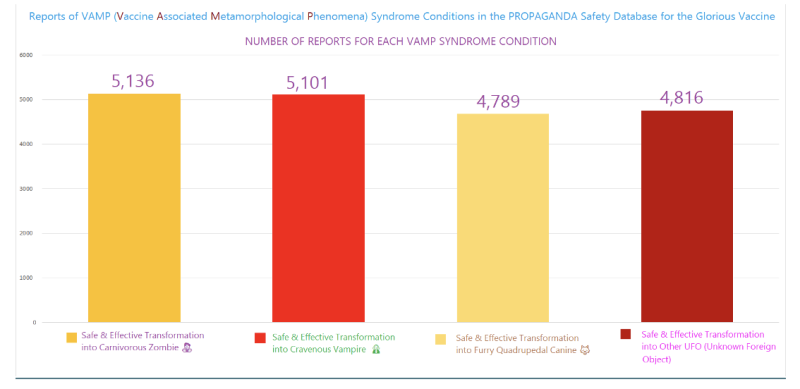
A patient comes in to the doctor’s office presenting with rapid, acute onset of Renfield’s syndrome (thirst for blood), extreme photosensitivity, pronounced macrodontia, and severe Contact Dermatitis to silver that all began within hours of getting jabbed with the Glorious Vaccine. This is an obvious case of a VAMP Syndrome side effect – the patient’s presentation fits the diagnostic criteria for fully-fledged vampirism and the condition was caused by the Glorious Vaccine (since you the doctor can safely rule out any other cause plus the immediacy of the onset of VAMP symptoms after getting jabbed is a pretty self-evident indicator that the Glorious Vaccine caused the symptoms).
Even though the patient can see that they are clearly not right – they feel an overwhelming temptation to chomp down on your pulsating jugular vein, they can’t stand being in front of a window unless the shades are completely drawn shut, they accidentally bit off a few pieces of their tongue with their newly extra-long and razor-sharp front teeth, and their skin starts peeling if they touch the silver family heirlooms – so what?? You can still tell the patient, “This is in your head” and send them home with a Xanax prescription (and maybe a bag or two of O-Negative blood if you sense that the patient might not be able to control themselves much longer and you don’t want your jugular to supply their lunch). And the patient will actually just accept it and go home without much of a fight.
This neatly avoids even generating any diagnostic record of VAMP Syndrome altogether, so there’s nothing to show up in any database anywhere.
You’d be surprised at just how many doctors are compliant to the point that they will convince themselves that the furry woman with a tail that grew out of nowhere an hour after getting the Glorious Vaccine has nothing whatsoever to do with the Glorious Vaccine.
(Note: In all seriousness, it is important to come up with catchy acronyms or names for things that convey the impression of how you want people to view the thing, so don’t use this example in real life, because it conveys that you are not taking safety surveillance seriously, and makes people more likely to believe that you are trying to hide the actual safety issues with the Glorious Vaccine.)
II-2. Over-Diagnose or Over-Identify Something
Conversely, if you need to make more of something than there is readily available, simply reverse #1. For instance, if you need people to be more fearful of the Dreaded Disease, you can implement a mass-testing regime to juice up the number of ‘confirmed’ cases of the Dreaded Disease. Also make sure to use tests that will return very high rates of positives, whether they’re true or not.
By increasing the surveillance or testing for something, you can generate the appearance of increasing numbers of whatever you’re testing for, or at least maintain the façade that it’s still around. Consider the following illustration from the good old US of A – you can see on the top chart that as the number of daily Covid tests went up, at the same time that the percentage of tests that were positive plummeted more than 75% (bottom chart). What this managed to do was keep the case numbers relatively high (middle chart), so even as the percentage of tests that were positive went down >75%, the number of new cases only decreased about 25% during that same time period.
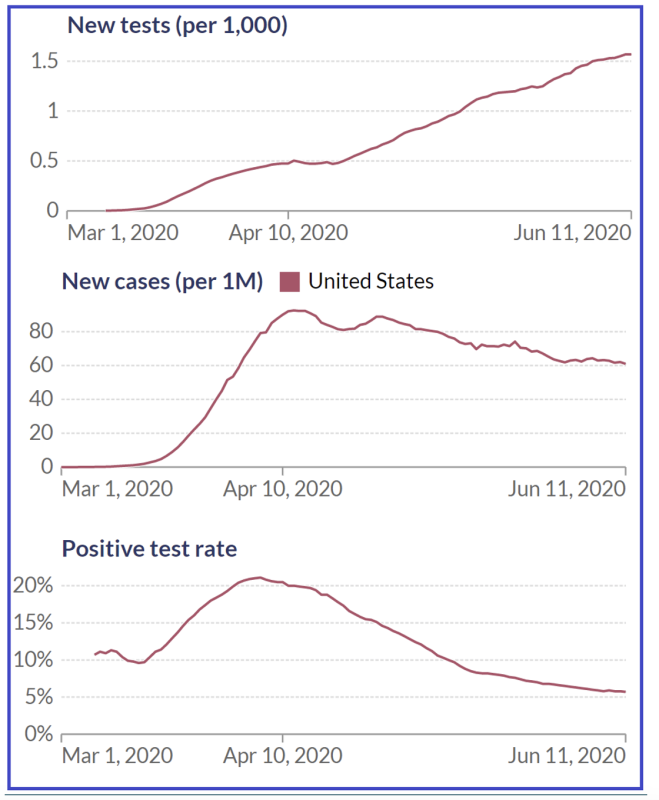
The meaningless increase in the raw number of cases that was entirely a function of more testing nevertheless resulted in headlines like this great NBC panic porn piece published on June 11, 2020:
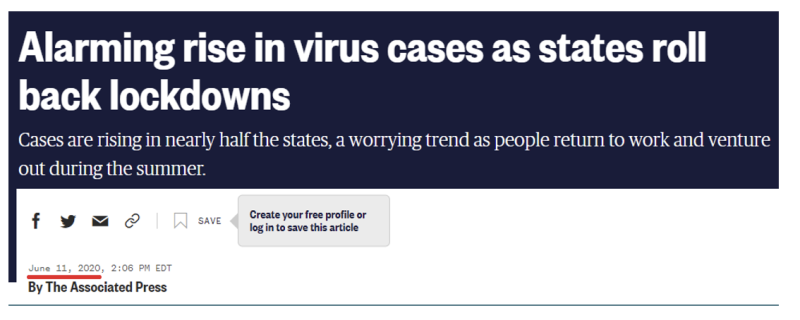
Remember: you find what you look for, and you find more of what you look for more.
II-3. Don’t Report What Is Diagnosed or Identified
Sometimes it isn’t possible to avoid diagnosing or identifying something best left undiscovered. In such a case, you can at least make sure that what was observed isn’t included in official reports or data:
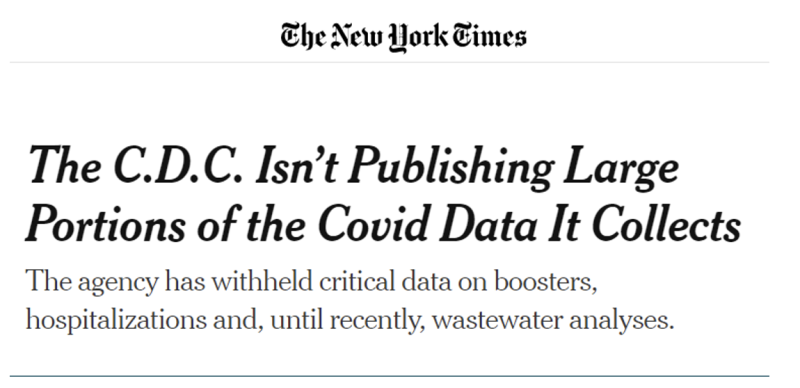
At a more individualized level, you should issue guidance to the doctors, medical personnel, and administrative staff on the ground NOT to diagnose things you don’t want showing up in datasets. Don’t hesitate to use financial incentives to sweeten the pot for loyal regime-compliant doctors. Don’t be stingy here – prevention is almost always cheaper (and less stressful) than fixing problems after they have already arisen.
Even in those rare cases where a doctor cannot avoid diagnosing a patient with a severe condition that occurred right after taking the Glorious Vaccine, the doctor can still make sure to avoid reporting the adverse event to any database of Glorious Vaccine injuries.
Alternatively, if the regime database for documenting injuries from the Glorious Vaccine somehow still ends up containing too many problematic reports where it is casting doubts upon its safety, there are two things you must do.
The first is to strand a few of the database administrators along the stretch of Somalia’s coastline where the pirates hang out so the rest of them get their act together and stop letting so many reports get through. You’re paying them to do a job, which is to maintain the public perception that the Glorious Vaccine is the safest drug product ever invented; failure is not acceptable.
The second is to NOT publicly disclose the problematic reports in the database. The CDC tried their darndest, but were ultimately defeated by a rogue judge (which emphasizes the need to have control over the judiciary as well):
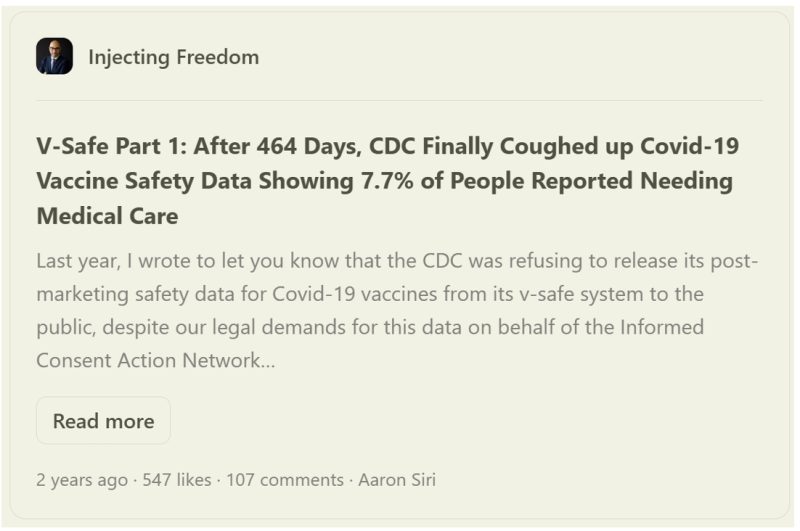
II-4. Don’t Allow Investigation of Phenomena if the Findings Might Cause Problems
The flip side of “You’ll find what you look for” is that “You won’t find what you don’t look for,” so make sure nobody goes looking for potential signals of something that could be problematic for the regime narrative. If, say, the regime ‘accidentally’ unleashes a plague in a third world city, you can’t have pesky social media conspiracy theorists figuring out what happened, so you best make sure that nobody conducts autopsies or tests sick individuals.

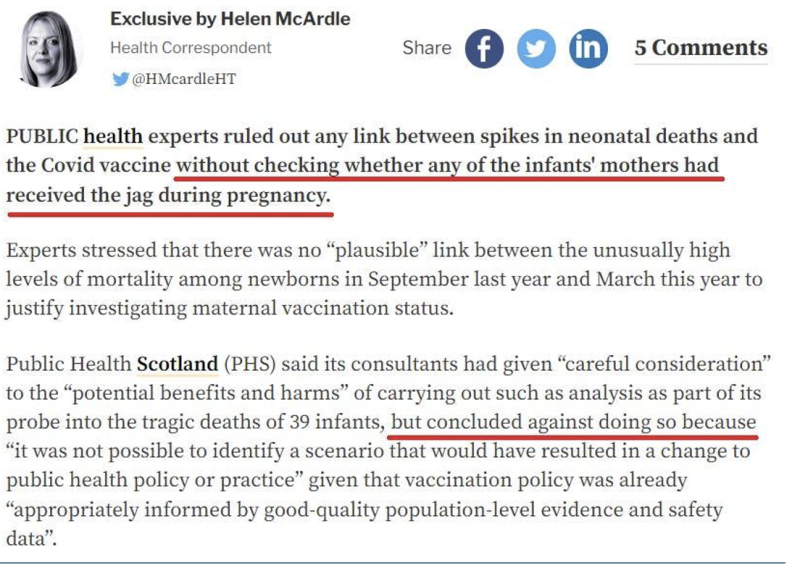
The CDC offers another illustration of good preemptive strategic thinking to keep out data potentially harmful to the regime:
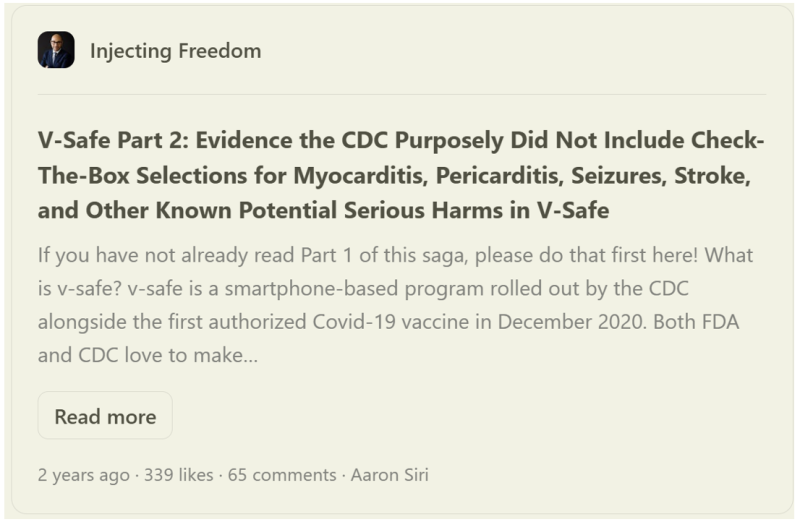
The CDC very smartly also has yet to commission a single autopsy of the thousands and thousands of deaths reported in the CDC’s own VAERS vaccine safety monitoring database. (Remember from Section I the part about adding absurd conditions to definitions? If you don’t, it’s best you review the material so that you have it on your fingertips.)
II-5. Publish only Part of the Data at First
Often enough, simply by publishing one part of the data and leaving out the other part for later, you can create a false narrative that takes root. So when you finally publish the rest of the data, it won’t matter that it contradicts the basis for what has now become accepted dogma.
For example, if you need to portray the Dreaded Disease as more widespread than it actually is, you could follow the lead of Virginia’s cutting edge propagandists and withhold some of the negative test results for a bit in order to raise the percentage of test results that are positive – which makes it seem like more people are sick with the Dreaded Disease:
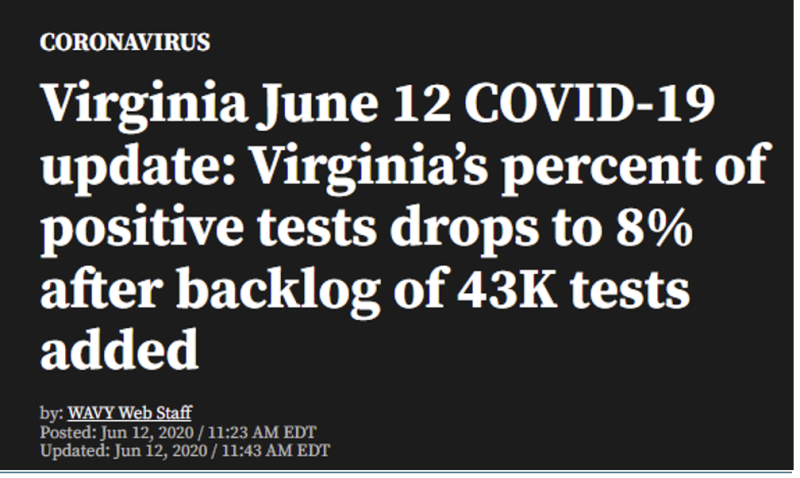
Another scenario where you can utilize the partial data publication technique to great effect is where you find yourself forced to release data for whatever reason that will make the regime look really bad (it happens). So you want to delay the release of the really damaging stuff for as long as possible – if you wait long enough, it will eventually cease being relevant. Also, if you dump it all at once, the shock factor will be enormous and you will have a big mess on your hands. However, if you release the information drip-drip-drip, then by the time the scandalous pieces are released, the “wow” shock factor has already long since worn off, and people are not going to be paying as much attention anymore. This tactic was attempted by the FDA, although it was mostly thwarted by the rogue judge (emphasizing the critical need for judicial control to prevent renegade judges from going rogue on the regime):
II-6. Limit the Acceptable Sources of Data or Information
When there are sources that generate data inconsistent with the regime narrative (it’ll happen once in a while despite your best efforts), simply discredit them as propaganda or something else uncredible and dangerous, like Russian bots. (As a general rule of thumb, you can always default to blaming or attributing any inconvenient information to “Russian disinformation” in a pinch.)
Exhibit A for this tactic would be the VAERS database run by the CDC. When VAERS showed utterly insane numbers of vaccine injuries by the Covid vaccine –
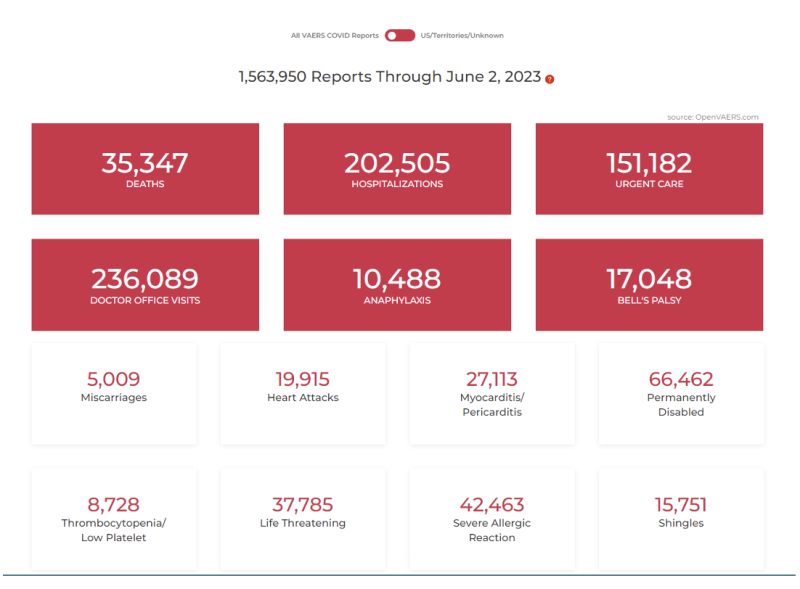
– the entire scientific establishment apparatus simply labeled VAERS as a conspiracy theory being used to spread dangerous disinformation:
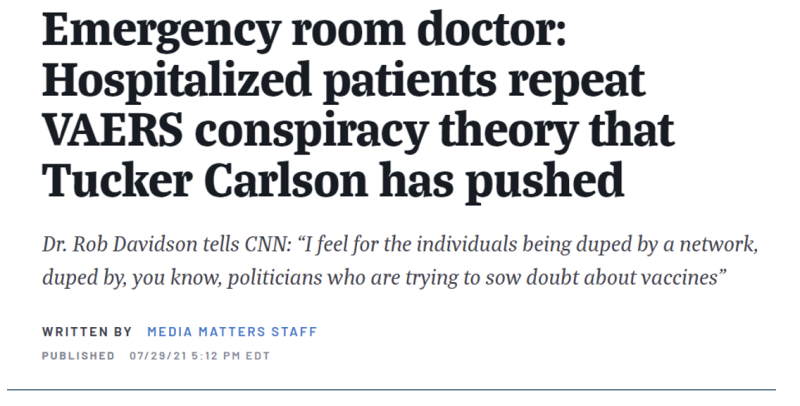
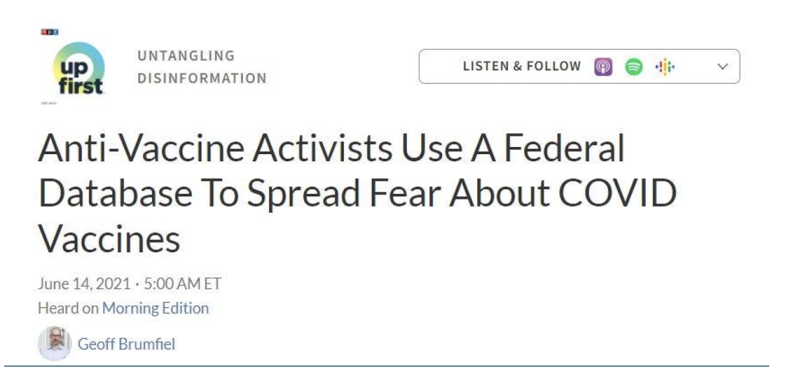
If, however, these data come from regime datasets that are too difficult to simply dismiss as unscientific garbage (yup, it happens), then stop publishing them and instead discredit them as being poorly constructed and rife with fatal errors.
We can use the UKHSA to illustrate this principle. After the crude vaccine efficacy dropped well into negative territory for almost all age groups (as in vaccinated people were at HIGHER risk for contracting Covid compared to unvaccinated people), the UKHSA simply stopped publishing the weekly vaccine efficacy data:
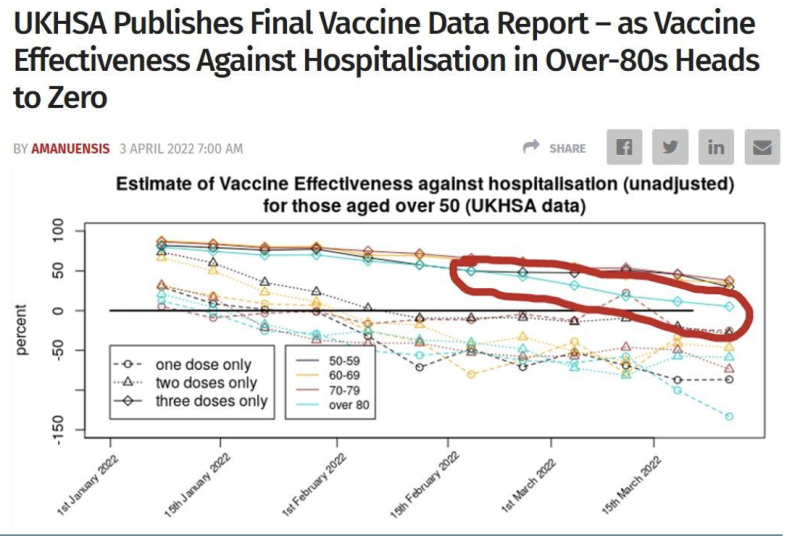
The UKHSA also offers a cautionary tale for what happens when you wait too long to pull the plug on problematic datasets:
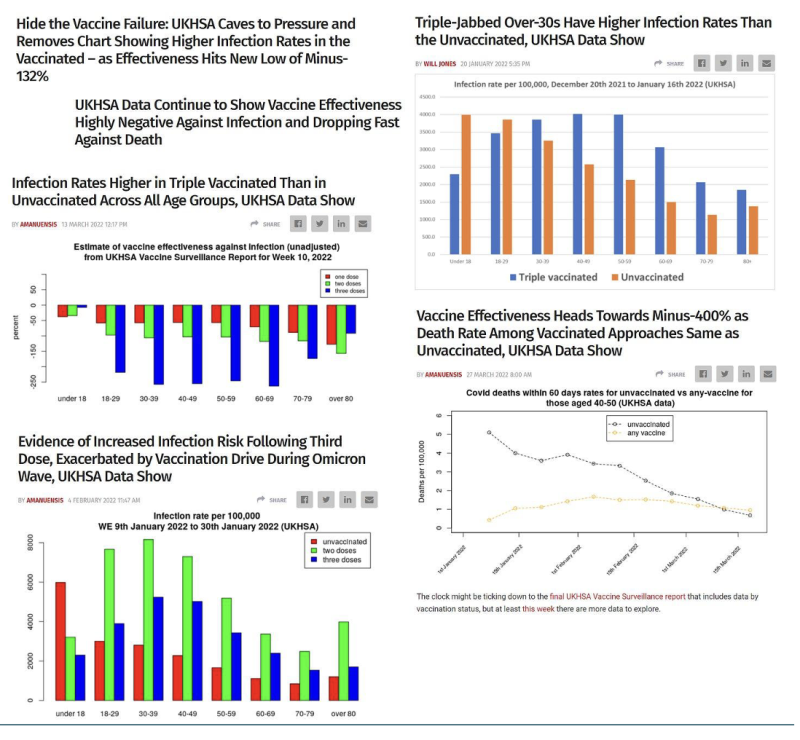
You can’t have headlines like these coming out every week!! They should have pulled the plug on this dataset waaaay before the vaccinated started getting Covid more than the unvaccinated. This is an unforced error, the kind of idiotic mistake for which heads roll, literally. Why on Earth did they wait until *booster* efficacy for the 80-year-olds was about to go negative???? Someone over at the UKHSA hasn’t read this book in a while, who clearly would have benefitted from a bit of review. . .
II-7. Employ Double Standards When Determining What Information Is Rigorous and Credible
Some propagandists may be hesitant to be nakedly hypocritical, because they feel exposed by openly postulating two irreconcilable standards that even some ordinary peasants can notice. You must fight this urge however. Understand that employing double standards exponentially increases your options when it comes to crafting talking points and positions for gaslighting the public.
This is particularly true when it comes to anecdotes. Anecdotes supporting the regime’s talking points, especially those from regime-approved sources, should be treated as the highest form of proof; whereas anecdotes from heretical or unapproved sources contradicting regime propaganda must be denounced as merely anecdotal and having zero evidentiary value that don’t count for anything at all.
So anecdotes from regime-compliant doctors and loyal citizens of the Dreaded Disease killing and maiming people are unassailable evidence, but anecdotes of injuries or deaths because of the Glorious Vaccine are nothing more than random coincidence if not outright fabrications, pushed by vile charlatans in order to defame the regime and endanger all good people everywhere who just want to stay alive and healthy:
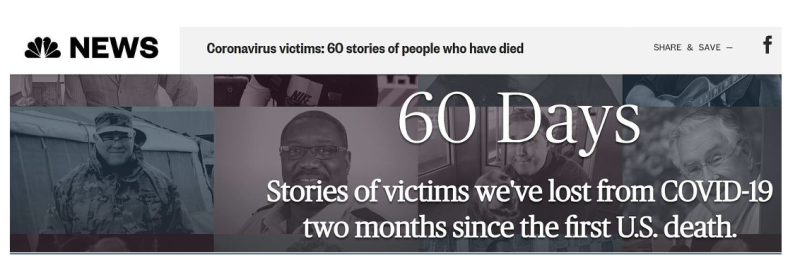

Openly employing double standards also has the added crucial benefit of conditioning the populace that the real standard for determining if data or information is reliable is simply what the regime says.
II-8. Corrupt the Data to Protect or Bolster Your Narrative
Sometimes, the easiest tactic to avoid problematic data is simply inventing fake data. You can fabricate something out of whole cloth. Or you can take a more nuanced approach, and corrupt the data by introducing subtle flaws or biases that are harder for the average person to notice. There are limitless ways to fabricate or falsify data, way too many to enumerate here. Just take care to falsify data in a way that is not easily discovered or reverse-engineered.
For instance, returning to our previous hypothetical situation where you need the population to believe that there are many more cases of a Dreaded Disease than there actually are, another way you can portray the Dreaded Disease as more prevalent is to combine the number of people currently sick with the number of people who are already recovered. The CDC actually did exactly this when they combined antibody tests (which measures the number of people who already recovered from Covid) with PCR tests (which measures the number of currently sick people) into one metric of “positive Covid test result,” deviously including everyone who already recovered as being CURRENTLY sick:
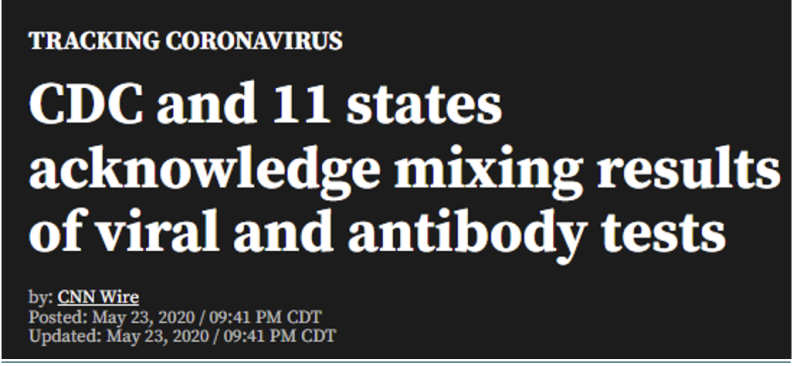
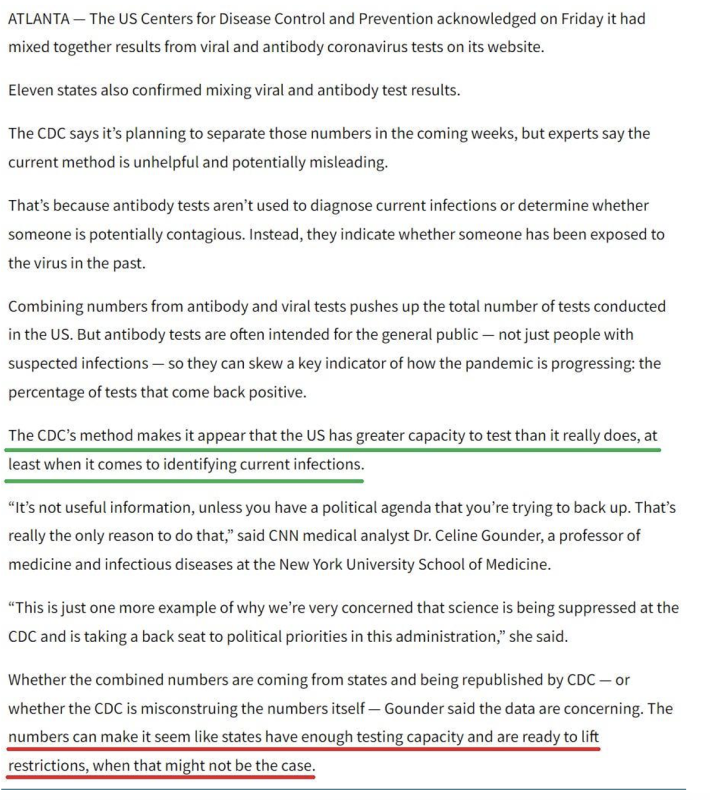
Notice the underlined sentences above, they are quite revelatory.
The green underlined sentence – “The CDC’s method makes it appear that the US has greater capacity to test than it really does” – observe how the CDC brilliantly managed to wrangle multiple propaganda cookies out of this one maneuver. Not only did they create the mirage of vastly higher rates of actively infected people; they also created the mirage that the government had a much greater capacity to test people for the virus than the government actually possessed. (It’s good to flaunt instances of government competence given that the government’s legendary reputation for staggering incompetence is one of the most notoriously difficult conceptions to push back on that people have about government.) A sharp propagandist is always looking to exploit additional angles for advantages instead of being content that the deployed propaganda tactic accomplished its primary intended objective.
The red underlined sentence – “The numbers can make it seem like states have enough testing capacity and are ready to lift restrictions, when that might not be the case” (and really the last two paragraphs) – offer a sagacious lesson about nipping potential thorns in the bud. You must always – always!! – be vigilant to head off *any* potential implications or quick-takes on information that even though is generally supportive of the regime, also contains something that can be twisted to undermine some other aspect of the official Regime narrative. Basically, you can have your cake AND eat it too! Appreciate here how the regime scientist quoted adroitly manages to simultaneously (1) voice approval of the increased testing capacity as a measure of the regime’s extraordinary competence; (2) lays the blame for the [intentional] “accident” upon the dissident political party; and (3) cautions that even though the state is doing such an awesome job making testing widely available, that doesn’t mean that it’s safe to reopen! Remember, there’s a pandemic to maintain, which this regime scientist expertly does. (Make sure to handsomely reward regime scientists for outstanding work like this. It’ll incentivize the rest of them to up their game and it’s good for morale.)
Also observe that the media is a critical regime ally without whom you will fail. So do what you gotta do to maintain the cozy relationships – don’t start penny-pinching here.
II-9. Delete Problematic Data
Yup. Like Bleach Bit-ing Hillary’s emails. It’s good to purge databases from time to time of data inconsistent with the regime’s narratives or positions; otherwise it might accumulate into a discernible trend that could be noticed by regime dissidents or disinformation spreaders.
So if for instance the safety database for the Glorious Vaccine contains too many reports, simply expunge them, like the CDC does, as illustrated by the chart below showing the number of problematic VAERS reports expunged by the CDC each week:
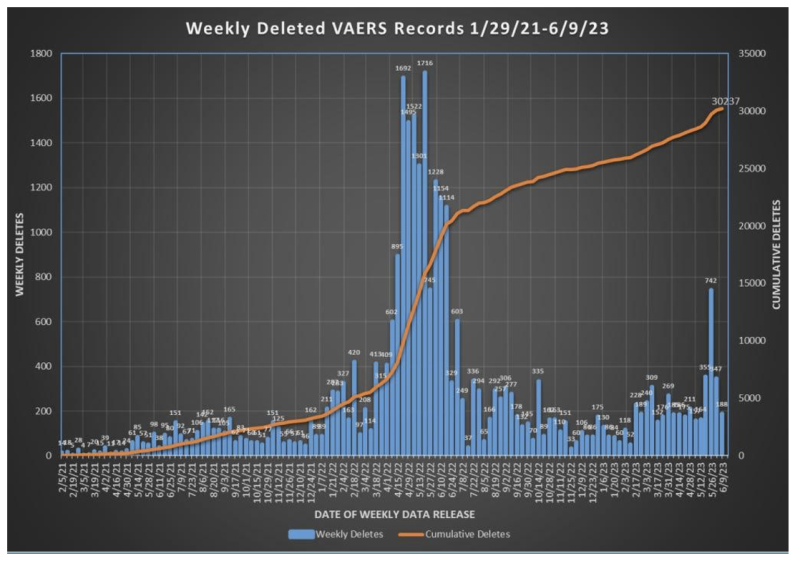
Notice in this instance that the CDC’s VAERS workers were underperforming for most of the time – you can’t allow critical personnel to slack off. This entire chart should show bars up to the top – there’s no valid reason why they couldn’t have deleted lots of VAERS reports in August 2021 like they did during April and May 2022. If you have to hire extra personnel to handle expunging reports, do it.
Also, why did these slackers allow so many reports to accumulate in the first place?? There shouldn’t even be enough reports in such a database to begin with where there would then be a need for weekly mass purges of said reports.
Perhaps the most important lesson of this entire book is this: The boring, droll, tedious logistical minutiae of disseminating and maintaining propaganda are every bit as critical as a grand sweeping Big Lie or breathtaking linguistic gymnastics.
You might have to get creative to come up with a justification or explanation for this if people figure out that there are missing data, so be sure to have talking points prepared in advance just in case.
Another great example of devious data deletion in action is the following brilliant expungement undertaken by the Australian government to eliminate inconvenient climate data showing heat records that happened too many years ago to be blamed on human carbon emissions:
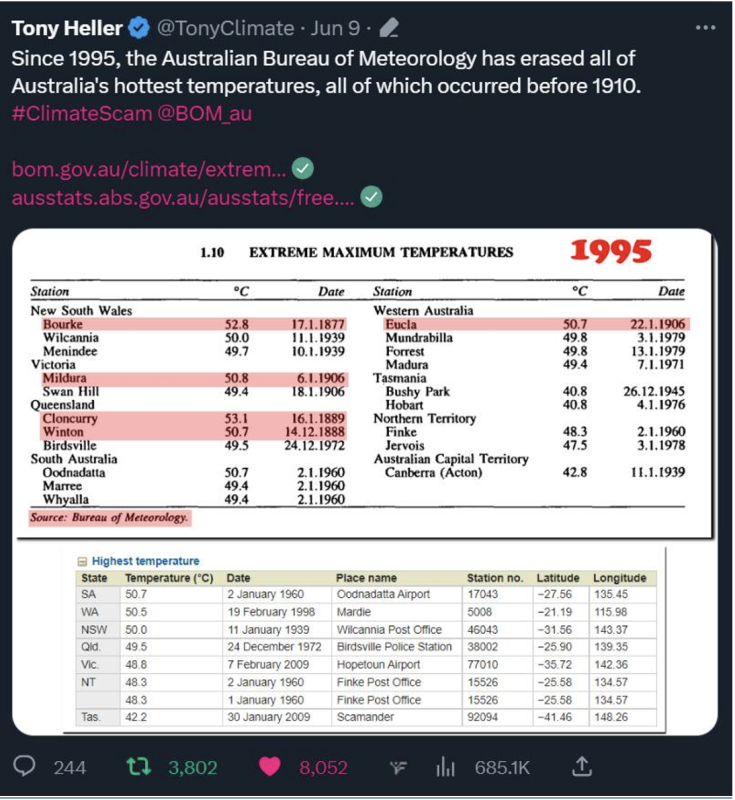
Unfortunately, they got busted, which is sometimes inevitable when you try to delete something really significant and noticeable. Which is why it’s imperative to have a Gulag system ready and waiting around the clock to handle a sudden surge of new inmates at a moment’s notice (like Australia’s quarantine camps).
II-10. Create False Data That Seems to Debunk Your Own Narrative to Trick and Discredit the Opposition
When confronted with a persistent information threat that is corrosive to your propaganda efforts, this is a brilliantly devious tactic to defoliate them of their authority, credibility, and clout. Simply put out false data that superficially seems to debunk the regime’s narrative, but that is easily disproved. The craven enemies of the state will undoubtedly seize upon this false info or data, and will therefore become discredited when you demonstrate that they fell for the now-obviously ludicrous claims.
Such as what the military did with their own in-house database of all the medical conditions for the entire military called DMED. They deliberately seeded it with fake data that looked like a total OMG!!!!!!!! moment that showed unholy massive increases in all sorts of medical conditions like cancers, pregnancy loss, and others associated with the sacred Covid vaccines. Then when a few heroic military doctors found the DMED data, they fell for it hook line and sinker…which killed the whole story. (For a full detailed timeline and explanation of this, see here.)
II-11. Use Pictures, Memes, or Other Types of Media to Lie Boldly and Audaciously
Much of public perception of the Science or data comes down to the visual presentation of the science or data – a good meme or image can effectively communicate completely false data in a way that leaves people with the conviction that the false data is absolutely 100% true.
For example, if you want to portray that the rates and severity of myocarditis caused by the Dreaded Disease is dramatically worse than the rates and severity of myocarditis caused by the Glorious Vaccine even though the precise opposite is true, you could create a powerful image like this:
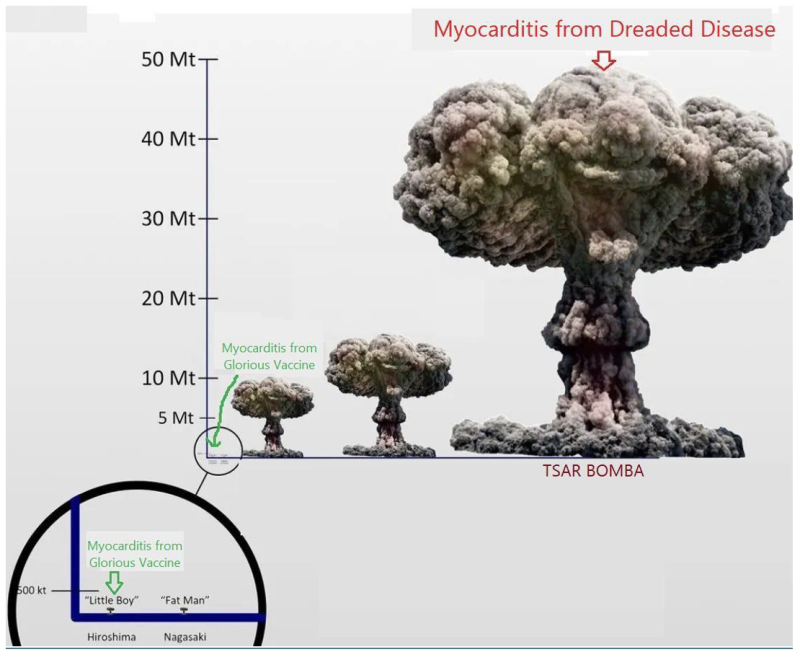
Now, people will instinctively associate “Dreaded Disease Myocarditis” with a massive mushroom cloud apocalypse vs myocarditis from the Glorious Vaccine as a tiny nothing pinprick that doesn’t even show on the chart.
II-12. Create Data Visualizations That Misrepresent the Data
Sometimes you can’t help but publish data that is really, really bad (for the regime or Science™️). But luckily for you, most people (and academics) are shallow idiots who are too lazy to read words printed next to a chart or graph. So you can deviously portray the data in a visualization scheme that distorts or hides what the data says.
Let’s illustrate using an example from the greatest of the Science™️journals – the Lancet. The Lancetpublished a study estimating the number of deaths caused by extreme cold and extreme heat around the world each year. Because governments around the world want to maintain the fiction of Global Warming being a mortal peril to humanity, they needed to show that deaths caused by heat outnumbered deaths caused by cold. At minimum, they had to be equal. Thus when the Lancet discovered that cold deaths outnumbered heat deaths by a 10 to 1 margin (literally), they had to figure out a way to create a chart that disguised that inconvenient little fact. Which resulted in the chart below on the left side:
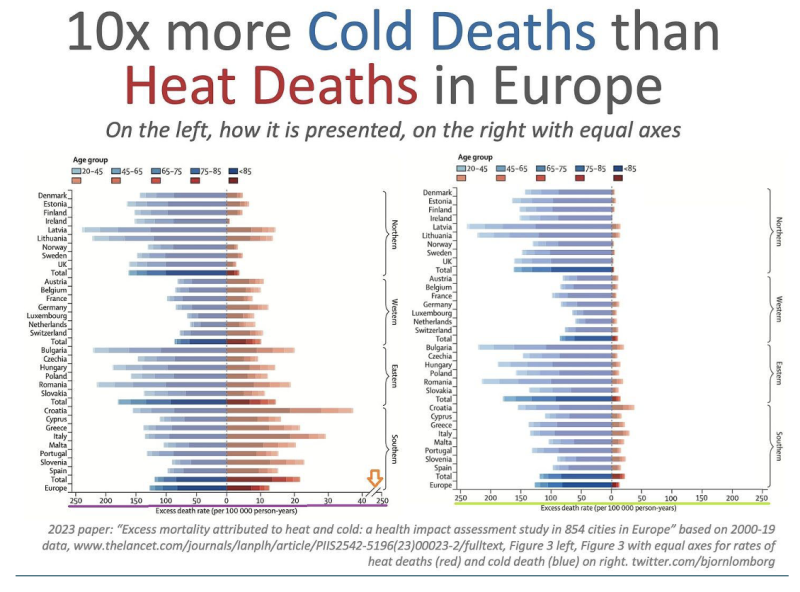
The blue bars show deaths from cold, the red bars show deaths from heat. The bigger the bar, the more deaths. So they needed to make the red bars be as big as the blue bars. So they employed a devious little trick – if you look at the purple underlined numbers which translate the size of the bar into a specific number of deaths, you’ll see that for the blue bars (cold deaths), each inch of bar represents 50 deaths, but for the red bars (heat deaths), each inch of bar represents only 10 deaths. Thus the same size bar represents 5x the number of deaths for cold deaths as it does for heat deaths, even though they look the same. But people don’t pay attention and will just go “Oh they look about similar so there must be a roughly equal proportion of heat deaths to cold deaths .” (And they even tried to sneak in a giant interval at the end where the last inch of red bars represents 210 deaths instead of only 10 (orange arrow).)
Had they created an honest chart that used the same scale for both cold deaths and heat deaths, it would look like the chart on the right. Thing is, one glance at that chart gives you the distinct impression that extreme cold is a vastly greater threat than extreme heat, which could lead to some uncomfortable questions about whether maybe a bit of Global Warming would actually be beneficial to humanity.
Note: When using this tactic, try to be more subtle and discreet than the Lancet, where it was very easy for even a layperson to spot the sleight of hand.
Rigging the SCIENCE
“To this end, Lysenko began to “educate” Soviet crops to sprout at different times of year by soaking them in freezing water, among other practices. He then claimed that future generations of crops would remember these environmental cues and, even without being treated themselves, would inherit the beneficial traits.”1
Rigging the science is nothing new. Fortunately for the propagandist, Science is very easy to manipulate at will if you are the regime. Just look at the accomplishments of Trofim Lysenko when he had the backing of Comrade Stalin. The following sections will detail what you need to do to successfully rig the Science to support the regime’s narrative and objectives.
A perfect illustration of a concerted and effective enterprise of Science-rigging is Big Pharma’s well-oiled propaganda machine. A group of renegade scientists conspired together to articulate precisely how Big Pharma controls and manipulates Science and Data at will:
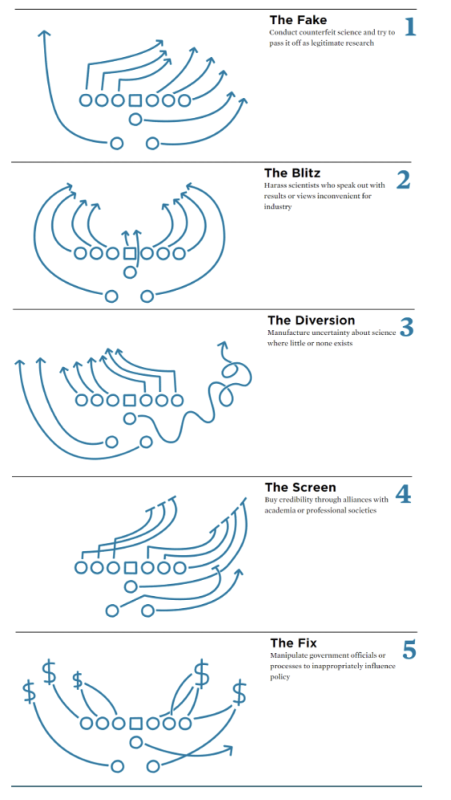
Obviously, the fact that this article is still publicly accessible is an astounding failure of the regime censors. In a country with a functional government, all the authors of such an audacious attack on the regime (and censors who failed to stop it from being published and/or didn’t take it down) would be deported to the North Pole, yesterday.
Sidebar: These authors do accurately describe how we corrupt the science to fit the regime agenda. Articles such as these, while they obviously cannot be allowed to disseminate publicly, are perfectly acceptable to disseminate among regime propagandists in order to better understand how to effectively propagandize.
Also important to note is that Pharmaceutical companies – “Big Pharma” – are usually going to be regime-compliant, but if a Pharma company becomes ‘less’ compliant, then you of course should prosecute them for their dastardly fraud. Also make sure to fine loyal Pharma companies big bucks every few years so the population thinks that the Regime has an adversarial relationship to Big Pharma and will therefore be less likely to realize that the regime and Pharma are in cahoots. A few billion is no big deal to their balance sheets.
Section III – Vetting Which Data Are Considered to Be Official Science
Be selective about which data are included in Official Science. Information that has the imprimatur of being Scientific information carries far more weight and credibility with the population, even those who refuse to toe the regime’s narrative (no one wants to be seen as being “anti-science” – that’s almost as bad as being a racist in modern society).
III-1. Don’t Publish Problematic Studies, and if They Get Published, Retract Them
The surest way to prevent official Scientific research from taking down a regime narrative is to strip it of its officialness. (Then you hide it away where nobody can access it and claim that since it was retracted that shows it was all along bogus fraudulent junk science pushed by corrupt Anti-Science heretics who want to get rich selling weird vitamin concoctions.)
You must take care however to act promptly, for if you wait too long, copies of unapproved science can circulate in secret amongst non-believers or heretics against the regime and take on nigh-mythical status. And once a study becomes entrenched in people’s experience as a “real study,” retracting it just makes them think that you’re desperate to hide the “truth.”
Take a look at all of these glorious retractions of studies that were harmful to the regime narrative during Covid (this is only the first page of 36):
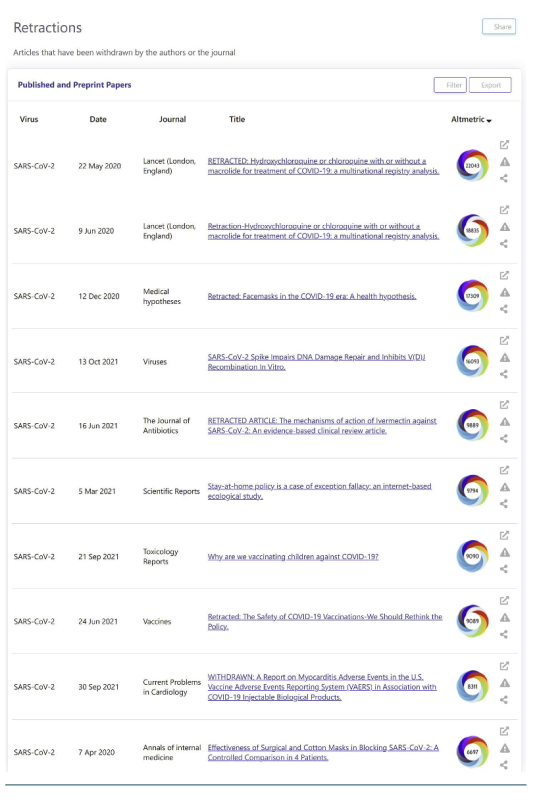
Imagine how much (more) damage these rogue studies could’ve done had they been allowed to remain and not been retracted!
Also imagine how many more studies never saw the light of day in the first place, since these represent only a small fraction of heretical research (or Good Science that accidentally found heretical results).
III-2. Cherry-Pick Which Parts of a Dataset Represent “Official Science”
It is amazing how drastically you can change the Science by simply utilizing selected portions of a dataset that bolster the regime narrative while discarding (or better yet, hiding) the parts of the dataset that are not in sync with the regime’s positions.
For example, let’s suppose that we see the following two trends in the regime’s PROPAGANDA safety monitoring database for the Glorious Vaccine.
(Unfortunately, you have to pretend to be monitoring safety to assuage jittery citizens who feel nervous about anything new, and also in order to have a ready-made response to potential critics and disinformation spreaders who will try and accuse the regime of hiding problematic safety data. And you have to pretend to take this VERY seriously.)
Anyhow let’s suppose there are 26,878 reports about Safe and Effective Transformations into Flesh-Eating Zombies per million doses of Glorious Vaccine administered, but only 2 reports about vaccinated people getting killed by flesh-eating bacteria right after being vaccinated, like this:
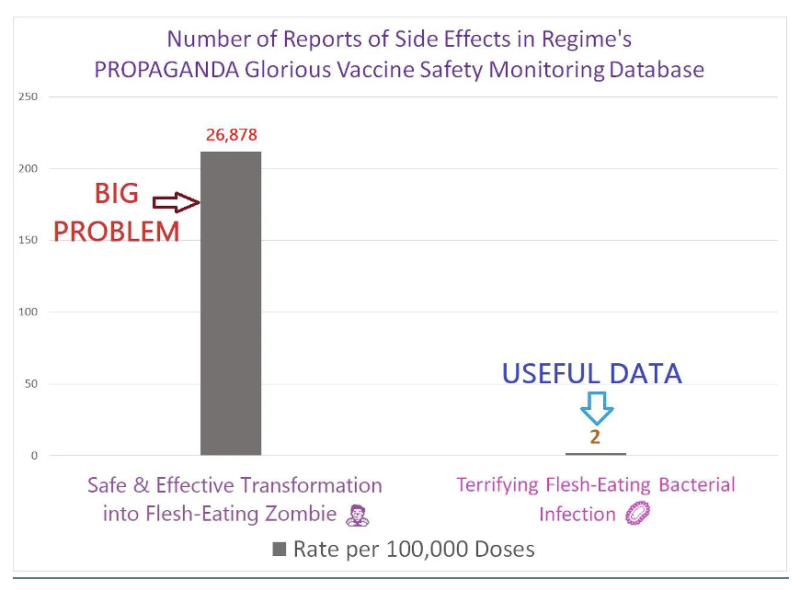
You can’t exactly have this get out into the public discourse, which will encourage vaccine hesitancy and cause people to doubt the regime narrative generally, even about other things. But you also need to demonstrate that the PROPAGANDA database shows that the rates of potential Glorious Vaccine injuries are negligible. (Make sure to emphasize whenever you refer to the safety database that these reports are not confirmed that the Glorious Vaccine was the cause, just a potential association.)
The solution here is quite simple – only use the data showing that there are only 2 reports of someone getting infected with Terrifying Flesh-Eating Bacteria because of the Glorious Vaccine per 100,000 doses. The 26,878 reports per 100,000 Doses of Safe and Effective Carnivorous Zombie Transformations, however, are to be publicly ignored as much as possible, and when you cannot avoid ignoring it, you must denounce it as unvetted unscientific and therefore meaningless reports that are therefore insignificant. And make sure to berate the media for daring to ask you about it. (Ideally you should conspire with a loyal Regime journalist that he should be the one to ask about it, so that it can be brought up in a dismissive manner like, “Some fringe people are trying to claim that the Glorious Vaccine is causing tens of thousands of sensational injuries, can you explain how they’re distorting the reports in the PROPAGANDA database?”)
Also, never use the word “terrifying” in the context of a situation where you are trying to calm people down. Never. Even if what you’re describing is objectively terrifying. When describing something that is inherently scary, instead use big, academic Sciency-sounding words. So “flesh eating bacteria” can be described as a ‘necrotizing fasciitis,’ something that nobody has any idea what the heck it means (and most people are too lazy to even Google it to find out). It even has two ‘i’’s in it, which makes it sound kind of impressive in an intellectual way, like it’s practically a privilege to be killed by something so sophisticated:
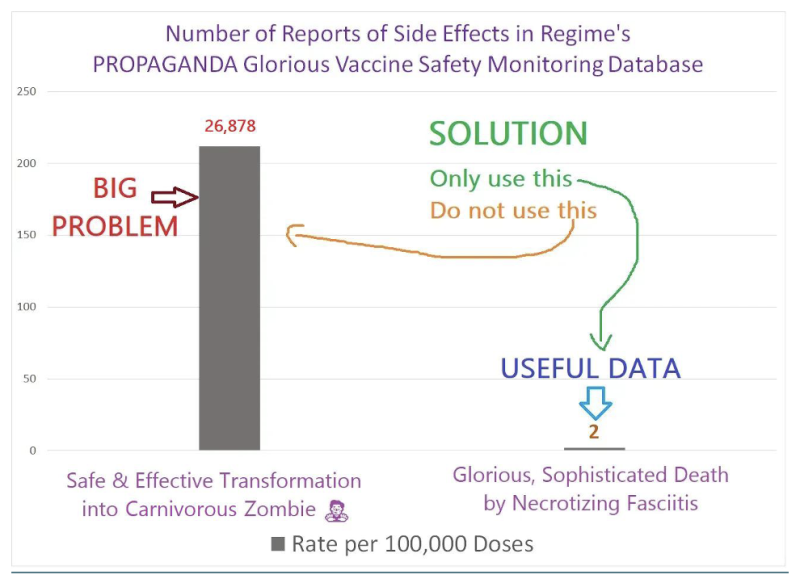
That’s not so complicated; you’ll get the hang of it in no time. (And if you don’t, you probably won’t be around for much longer anyway.)
Note: When you have a situation where a regime-endorsed or mandated product is dangerous – **which will be often** – you must make sure that you do not fall for your own propaganda; otherwise you might well end up the next Safe and Effective Zombie like these four US senators:
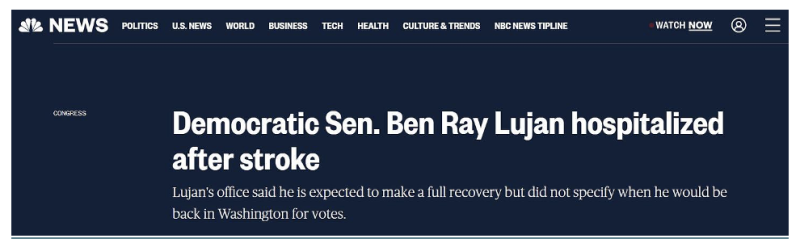
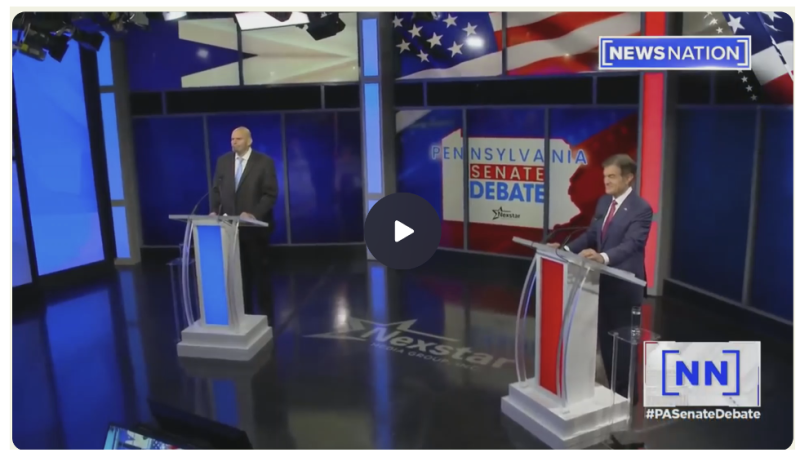
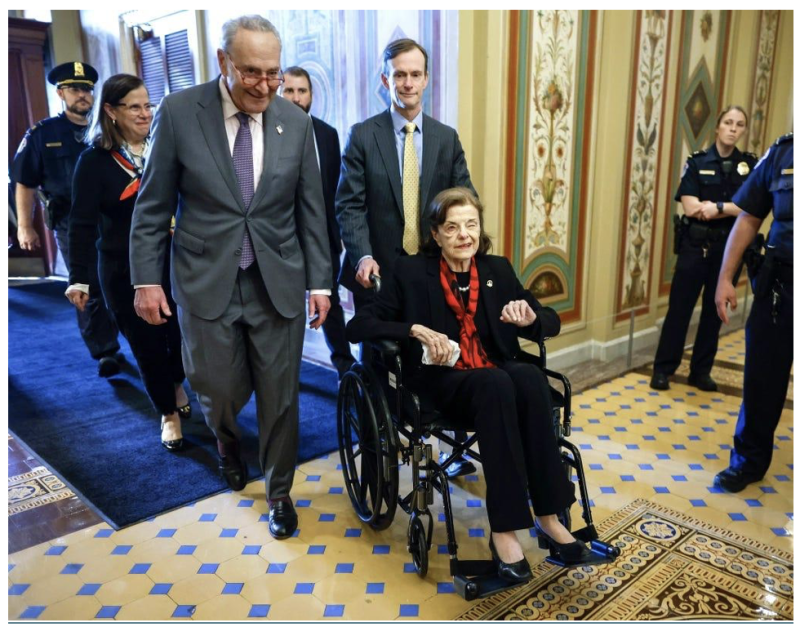
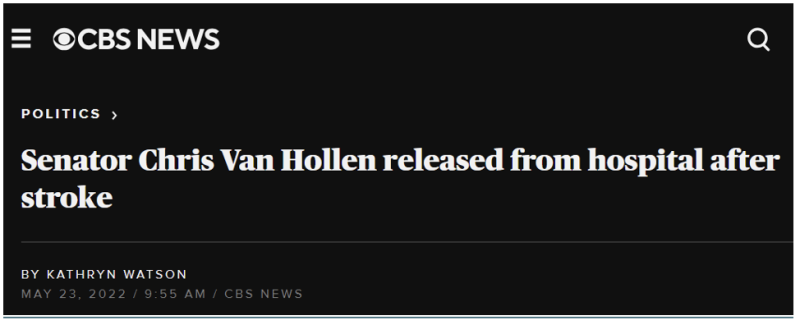
III-3. Delay Reporting Data
A more subtle way to vet what data is included in the Official Science is to dishonestly report data or information. Strategically timing the reporting of different subsets of data is a simple yet powerfully effective way to manipulate the scientific data. (Don’t worry about understanding how this works; just know that it does, and hire competent statisticians who can figure out how to best implement this.) Many calculations rely upon the timing of the reported data, and you can therefore control what the data show by carefully releasing different portions of the data at the optimal time.
For example, a one-week delay in the reporting of deaths can radically change the apparent efficacy or safety of a medical intervention – literally, by delaying the reporting of deaths by a week, you can make something that has zero effect look like it’s 95% effective. (You can follow the link for more details, but this particular tactic is a bit too complex for an Idiot’s Guide, and including an in-depth description here might cause otherwise budding propagandists with a brilliant future ahead of them to get depressed and doubt their own abilities if they can’t follow the explanation, which can lead them to quit, which would be a tragedy. Truly.)
Section IV – How to Rig a Study
Perhaps the most crucial skillset necessary to manipulate Science is the ability to design and manipulate a study to achieve the necessary results.
[Note: the actual rigging of studies will always be done by experts who run studies for a living (called PI’s, or Principal Investigators). So you don’t really need to be fluent in this stuff. But it is nevertheless helpful to have a decent enough grasp of the basics.]
Studies – especially the big fancy ones that are typically considered to be the “gold standard” of Science™️ – are massively complex beasts that can be manipulated in innumerable ways. We will explain the more prominent and straightforward types of deceptions, manipulations, and design flaws that can be exploited to make the study a puppet in your hands to jerk around at will.
[Note: – there are many gradations of sophistication in implementing any of the following manipulations. We’re only going to explain and illustrate the underlying concepts using the straightforward plain application of the principles, without adding any fancy adornments and baubles. The goal here is that you should understand the various types and ways to manipulate data. You can educate yourself in the more advanced methodologies afterwards (something which is of course highly encouraged and recommended).
IV-1. Study Rigging Tactic #1: Rig the Design of Study Protocols
Most of the material relevant to this section is also relevant to the next section dealing with sabotaging the implementation of the study protocols, so we will only address here the tactics unique to rigging the design of the protocols themselves.
Study protocols are basically like a rulebook dictating how the study is going to be done. So make sure to write rules that favor the outcome you need to get.
A) Stacking the deck – strategically assign study subjects to the respective study and control groups
Almost all big, special studies have two groups – the study group, and the control group. In a study for a new medicine, the study group gets the medicine, and the control group does not. In theory, if the medicine works, then there should be more sick people in the control group than in the study group.
So if you are running a study to test a new regime Wunder-drug, you could exploit this by putting more unhealthy people in the control group than in the study group so that the study group will do better even if the regime drug doesn’t work. (You should of course not admit to doing this or any other of these tactical shenanigans in study documentation.)
B) Carefully vet subjects to be included in the study
Much headache can be avoided simply by keeping out people who are likely to mess up your results in some way.
For example, if you’re testing a novel drug that you want to prove is safe and effective, keep out people who are particularly disposed to suffer bad reactions or inefficacy. You get the idea. (Like they didn’t include any old comorbid people in the Covid vaccine trials, which would have exposed the ‘99% effective’ canard.)
IV-2. Study Rigging Tactic #2: Sabotage the Execution of the Study Protocols
Often enough, you will not be able to rig the study protocols themselves outright to produce your desired results. In such cases, you need to sabotage the implementation or adherence to the official study protocols instead. This is quite easy to do, and there are literally endless ways to accomplish this.
Note: It is prudent to have your logistics planned out in advance, in order to avoid a variety of problems and stressful situations that can pop up in a big study involving thousands of subjects and personnel. For instance, if you want to ‘show’ that a particularly annoying drug is actually lethal, you should have body bags on hand to quickly remove bodies from public locations and a cremation facility on call 24-7 to destroy any undesirable forensic or pathological evidence that corpses may contain.)
Protocol Sabotage #1: Administration of the study treatment/intervention [to the study group]
People think that giving study subjects a drug is uncomplicated and straightforward. They’re wrong. Very very wrong. You can frequently control the entire study by subtly adjusting how the treatment gets administered to the study subjects, including the following:
- Dosing/Quantity of intervention – You can underdose or overdose a drug depending on what you’re aiming for. If you want the drug to look ineffective, underdosing will ensure that it won’t work. If you want to show that the drug is dangerous, just amp up the dose to highly toxic levels.
- Timing of treatment administration – Another way to sabotage a drug is to give it to patients too early or too late to be effective. There are many different tacks you can choose to accomplish this. For example, you can send the drug to patients through the mail, which will inevitably add a few days to the timetable (a David Boulware Ivermectin special).
- Quality of the product – i.e. purity/potency – A contaminated or poorly manufactured product will not function the same way a pure product manufactured with high-quality ingredients and complete fidelity to the ideal manufacturing practices.
(Note: You should ALWAYS conduct off-the-record pre-clinical studies on animals – and humans – to understand how different versions of the drug or intervention will function BEFORE deploying contaminated versions in a study (in addition to the official pre-clinical studies on the normal formulation of the drug); otherwise you run the risk of accidentally sabotaging your own sabotage attempts. Remember, the point of running the study is to show a pre-ordained outcome, not discover any novel scientific insights! Uncertainty or unpredictability about what the drug or intervention you’re studying will do in real life is Kryptonite to successful study rigging. Or at minimum is going to give you some really bad migraines while you struggle to navigate the maze of hazards and uncomfortable data from your now-extremely messy study.)
- Use saline or placebo instead of the intervention – Another way you can minimize hazards of the regime’s chosen intervention is to give a placebo instead of the treatment, so that there is less exposure to the toxicity of the intervention. Obviously, you need to also make sure that using saline won’t have the unwanted side effect of showing that your drug doesn’t work, so this tactic is typically used in conjunction with other protocol manipulations or infidelities.
- Mix and match – You can always mix and match within any of these suggestions. For example, you can give some of the treatment subjects a different product. You can also employ more than one of these suggestions in combination so that you cover different portions of the study group with different suggestions, which can make it harder for outsiders to discover the protocol violations.
Protocol Sabotage #2: Administration of the placebo [to the study group]
This is essentially the flip side of the previous section. There are a few specific tactics that are a bit unique as applied to the placebo:
- Give the control/placebo group the intervention – One way to guarantee that a study won’t show any efficacy for a treatment is to give the control group the treatment as well. If both groups get the treatment, then there won’t be a difference between them showing that the treatment group fared better because of the treatment.
The easier but riskier method of doing this is to have the study personnel directly give the drug to the control group masquerading as the placebo. (This is easy enough, because the placebo is supposed to look, feel, taste, and smell identically to the treatment to prevent the control group subjects from figuring out that they didn’t get the drug.)
The more difficult but less risky method is to nudge the control group subjects to obtain the treatment outside of the study. For instance, you can use a placebo that is markedly different from the drug. Since the study subjects can easily discover via Google that this isn’t what the drug is supposed to look, smell, or taste like, they will endeavor to procure the actual drug on the side since they don’t want to die or suffer debilitating complications from whatever disease or condition the drug is being used to treat.
Alternatively, you can choose to run the study in a place where the population already has wide exposure to the treatment being studied, so the pool of subjects will be thoroughly contaminated with people already using or at least have a supply of the drug on hand.
(Just keep in mind that this tactic runs the risk of being noticed by pesky dissident anti-Science heretics, since it will be a matter of public record that there was widespread awareness and/or use of the drug where the study was conducted.)
- Spike the placebo – If you don’t want an inert placebo, you can spike it with something a bit more ‘lively’ that can elicit side effects and/or a therapeutic effect.
One specific method is to use components of the treatment to spike the placebo. This can be especially useful for hiding problematic side effects of a treatment that are caused by other ingredients or components besides the active treatment ingredient – if you put those in the placebo, then both groups will have similar side effects.
(Note: Keep in mind that if the side effects are too pronounced, simply putting the toxic components of the treatment in the placebo may raise questions if people notice that the rates of the specific side effects are vastly higher in the study’s control group than they are in the general population.)
Protocol Sabotage #3: Incentivize the study subjects to modify their behavior
The behavior of study subjects is often a critical consideration when designing protocols and running a study. Use this to your advantage.
There are 3 basic types of incentives:
- Financial inducements – One of the surest ways to incentivize a behavior is to reward it financially:
- You can run a corrupt bribery scheme within the study. For example, if the study is obtaining results by asking subjects to report information – such as what side effects they experienced after getting the Glorious Intervention – you can pay subjects to not report side effects. However, you also will have to enforce secrecy and ensure that nobody finds out about it, which can be tricky.
- Alternatively, you can manipulate or take advantage of the environment where the study is taking place to function as your intermediary or middleman to dispense the financial goodies. For example, if you are testing the effectiveness of a potential intervention to block transmission of the Dreaded Disease, you can run the study in a place where people can only go to work if they are not infected with the Dreaded Disease, taking advantage of this built-in incentive to not report testing positive that people have (they want their full paycheck).
- Social Pressure – The second type of incentive is social pressure. This can come from peers, political forces, social groups, professional associates, institutions, celebrities, or any other source of influence in society. The point is you can use any or all of these to your advantage.
For example, let’s say that you are running a study to test the effectiveness of the Wondrous Cloth Shield that stops the spread of the Dreaded Disease. So you give some villages in a third world country the Wondrous Cloth Shield, and create a control group of villages who don’t get the Wondrous Cloth Shield. You can make a show of how amazing these devices are in front of the villagers who get them. You can also have the village elders proclaim that the Wondrous Cloth Shield is a Gift from Heaven, which makes it a point of moral virtue to wear one, and more importantly, makes wearing one but getting infected with the Dreaded Disease a mark of religious failure. Which makes them far less likely to report cases of the Dreaded Disease, especially compared to the villages who weren’t given the Wondrous Cloth Shields. Which makes it look like the Wondrous Cloth Shield works to reduce Dreaded Disease transmission. - Harsh Penalties – You can threaten all sorts of dreadful consequences if study subjects don’t do exactly what you want. This is especially easy to implement in third world countries where there is little if any rule of law and corruption is the rule. It might be useful to make an example of someone in advance to show that you mean business – for instance, you can pick someone at random to ship off to a prison in Sudan, from which they are unlikely to ever return alive.
Protocol Sabotage #4: Hire incompetent people to run the study
Studies – especially the studies that perform some sort of experiment (as opposed to just analyzing pre-existing datasets) – typically require large staffs to conduct. Hiring incompetent staff is a great way to give yourself some leeway to “massage” inconvenient data that emerges from the study – “this data is erroneous because the staff messed it up.” So of course, you have to “fix” the “errors.”
More importantly, incompetent staff are less likely to notice that you’re rigging the study because they don’t have the knowledge or experience about how a legitimate study is supposed to be run.
Protocol Sabotage #5: Remove any problematic study subjects or events from the study
This one is an obvious “Duh.” If a few subjects in the phase-3 trial for the Glorious Vaccine suffer severe injuries right after getting injected with the Glorious Vaccine, well, you can’t have them ruining the “safe and effective” narrative. But thankfully, the solution is simple: remove them from the study.
This won’t even look suspicious to an outside observer! Every study has rules written into the protocols that allow you to kick out subjects who violate the study protocols or wish to leave for “personal reasons.” (Think of every time a politician says he’s resigning to “spend more time with his family” – same idea.) But most academics are suckers for this and fall for it every time.
If you’re really smart about how you design the protocols in the first place, you will add a condition that prohibits subjects from seeking medical care from any doctor outside the study. So if a subject suffers a nasty side effect, like a bit of safe and effective myocarditis or some mild Bell’s palsy that leaves him somewhat paralyzed, they’re gonna go straight to the nearest ER…which is a clear violation of the study protocols!! Bye-bye problem.
If you want to see a real-world maestro, look no further than the fellow in charge of Pfizer’s Phase 3 Kiddie trial for their vaccine – when one of the trial subjects by the name of Maddie de Garay suffered multiple rather nasty neurological injuries 24 hours after getting the vaccine (the sort that involves permanent use of feeding tubes and wheelchairs among other lifestyle ‘adjustments’), they simply threw her out of the study. And then wrote up her injury as ‘unresolved abdominal pain.’ They also threw out another fellow from the main trial, a lawyer named Augusto Rioux, after he got some mild safe and effective pericarditis following Dose #1.
Same for AstraZeneca – Brianne Dressen was chucked following Dose #1 – but they reported that she withdrew for personal reasons. See? Easy-peasy.
Protocol Sabotage #6: Record False Data
When all else fails, you can simply record data for the study that is dead wrong and fabricated out of thin air. Pfizer study contractor Ventavia shows us the way on this one – the following screenshots are the actual email sent by Brooke Jackson – one of Ventavia’s Site Managers – who decided to attempt to undercut the regime by exposing the ongoing fraud:
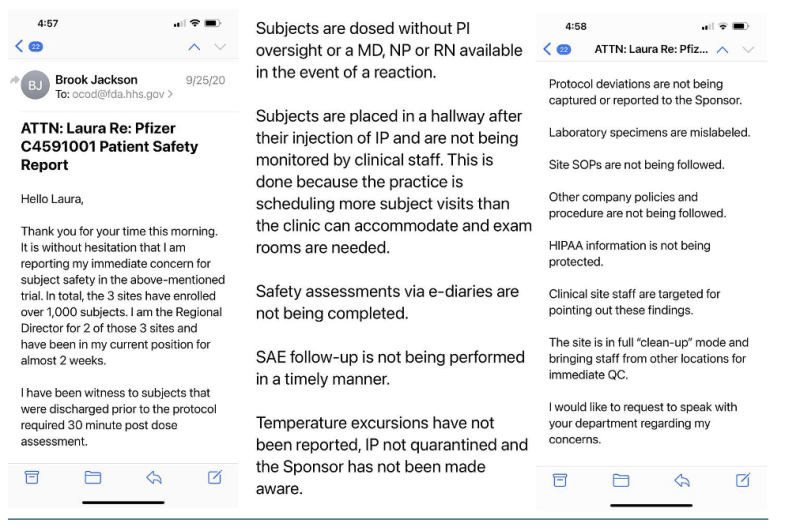
In an unusually prompt and effective response, Mrs Jackson was fired less than six – 6 – hours after sending the FDA this email. SIX HOURS!! That is how things should get done.
Furthermore, when she sued in Federal court in an attempt to bring down the entire Pfizer vaccine trial, the regime successfully stalled it for almost two whole years using a variety of ingenious legal tactics. (However, it should be noted that whoever was in charge of hiring blew it big time; you gotta do thorough background checks to make sure that prospective applicants do not possess strong moral convictions.)
Unfortunately, the FDA does not control foreign medical journals, one of which decided to (shockingly) publish an article documenting the Pfizer trial fraud. Big whoopsie. This is why it is imperative to establish a unitary governing body for the whole world.
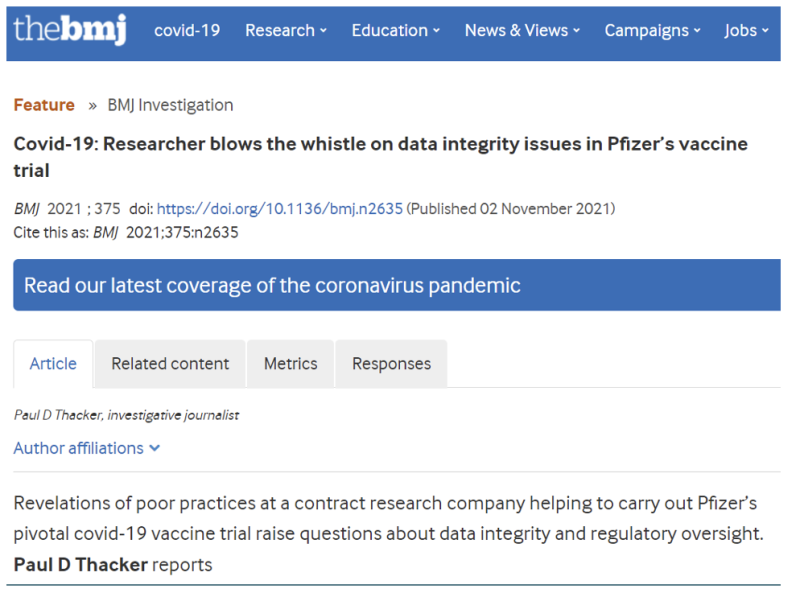
IV-3. Study Rigging Option #3: Study Analysis
Once you finish the study itself, now it’s time to crunch the numbers from the study. Any problematic data that somehow got through all your protocol designs and sabotage will be cleaned up here. Think of this like giving a used dinged-up car a brand new coat of paint to hide all the damage underneath – you’re not changing anything substantial, just disguising stuff (for the most part). Nobody wants to scratch the fresh new paint to make sure it’s not hiding something.
There are soooo many ways of ‘analyzing’ the data. The trick is to be smart about which ones you pick and how you go about doing the analysis.
Analysis Tactic #1: Don’t adjust the data
Data adjustments are pretty standard stuff in science. Raw data is almost never suitable for directly drawing inferences or extrapolating from, because there are usually all sorts of confounding variables present.
Here is a very simple example of a data adjustment:
The following is the population of the states of Darth Santistan (bad state) and The Gender Spectral Paradise of Commiefornia (good state):
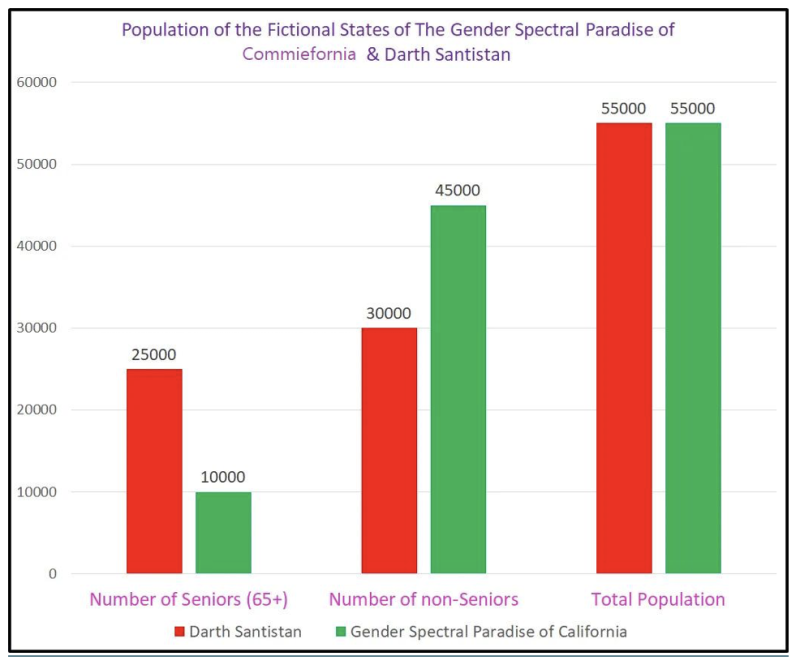
Here are the death rates from the Dreaded Disease for these states – overall, the bad state has more deaths than the good state. Since they have the same population, this means that the death rate is higher in the BAD, BAD state of Darth Santistan:
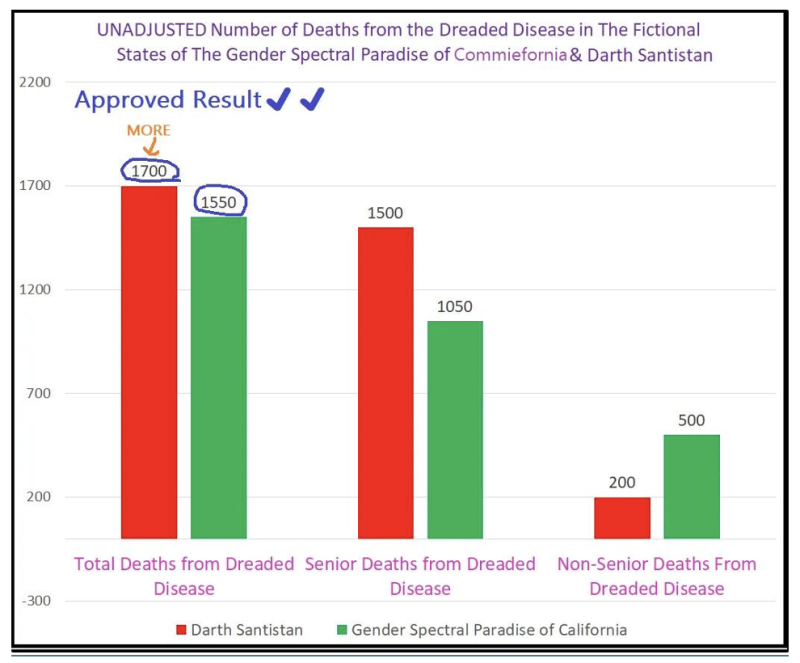
BUT. . . (yes, there is a big ‘but’ here)
If we look at the death rates for the senior population and non-senior populations separately, shockingly the good state has a higher death rate in BOTH (?!?!?!?!?):
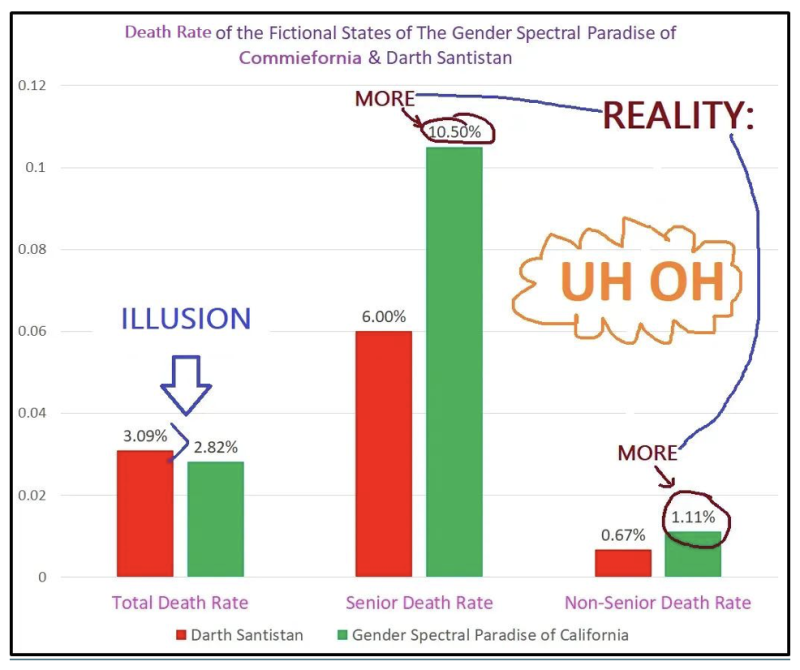
Two important observations here:
- The reason that the disloyal state of Death Santistan has a higher overall rate despite having lower death rates in each age cohort is very simple actually – seniors die far more often than non-seniors, but the bad state has the misfortune of having 2.5x as many seniors as the good state, which means a lot more deaths overall because of the sheer number of senior citizens in the bad state of Death Santistan:
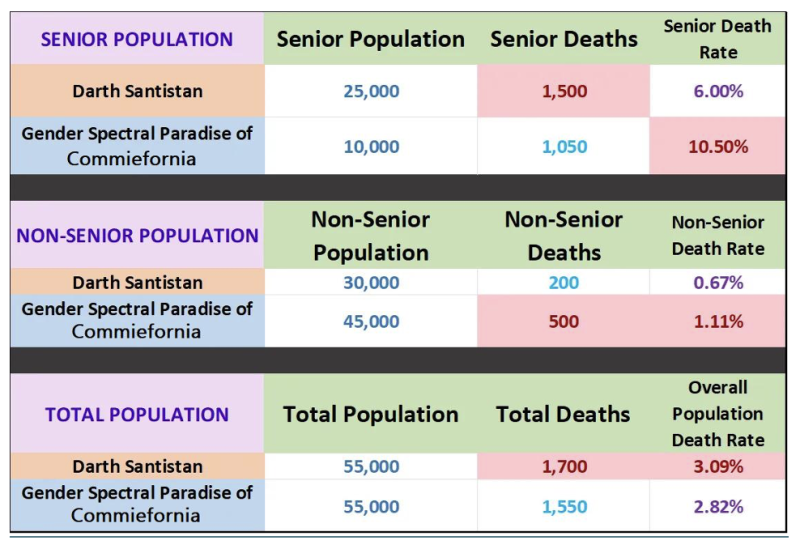
In order for the bad state to have the same number of senior deaths as the good state, they would have to have literally 40% of the death rate in seniors as the good state because the good state has only 40% as many seniors in their population as the bad state. This is why (when we want to be honest, like when the truth helps the regime) Science adjusts data – to avoid stuff like this. (This particular statistical phenomenon actually has an official name: “Simpson’s paradox.”)
Therefore, DON’T adjust the data when it will hurt the regime’s narrative.
Analysis Tactic #2: Adjust the data deceptively or inappropriately
Conversely, sometimes the raw data, or properly adjusted data, will not be good for your narrative. In such cases, you gotta keep adjusting in creative ways until you’ve successfully obscured the heretical results so nobody can see them or figure them out.
For instance, if we take our above hypothetical comparison of the fictional states of the Gender Spectral Paradise of Commiefornia/Death Santistan, you can add an ‘adjustment’ to “fix” the problem. All you need to do is find a characteristic that is a proxy for worse outcomes in the Bad State of Death Santistan than the good state of Gender Spectral Paradise of Commiefornia. Since Death Santistan decided not to follow the regime’s Lifesaving Lockdowns, the seniors in Death Santistan tended to leave their houses more than other states, even if just to walk around the block for fresh air — meaning that seniors who didn’t leave their houses probably were more often too sick to leave their house. Such sick seniors are also more likely to be the ones who die from the Dreaded Disease.
Here’s how this could play out:
Chart #1 – population of seniors in each state (left columns = seniors who went outside at least once a week; middle = seniors who didn’t go outside; right = total number of seniors in each state)
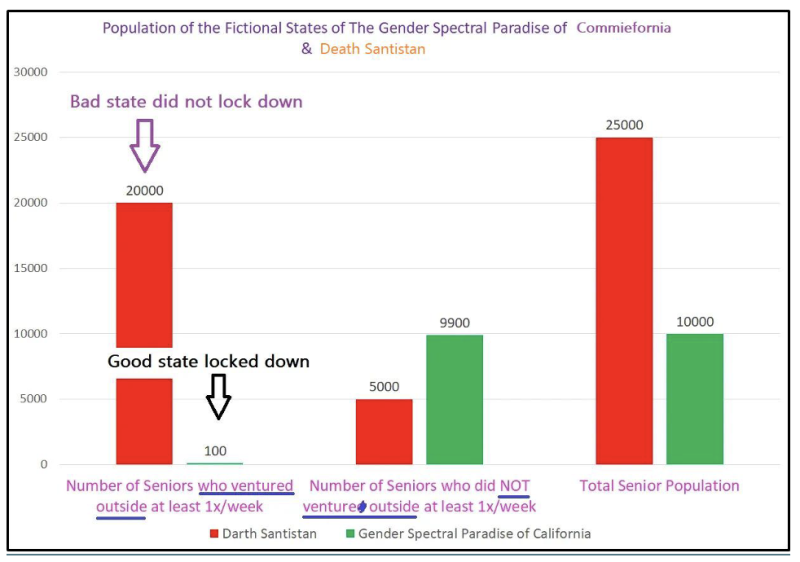
Chart #2 – number of deaths in each of the three categories in Chart #1:
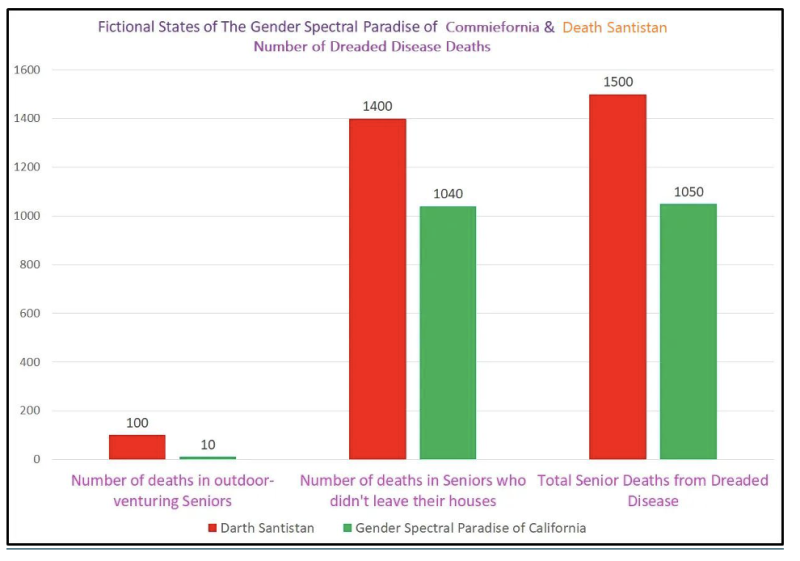
This completely fixes our problematic data (it might actually fix it too well!!) – observe how we are changing the death rate in seniors:
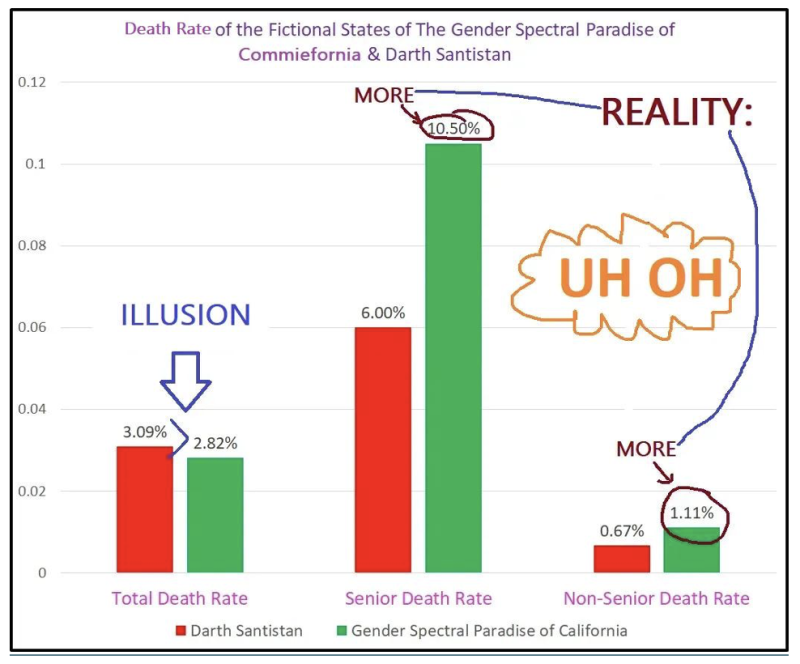
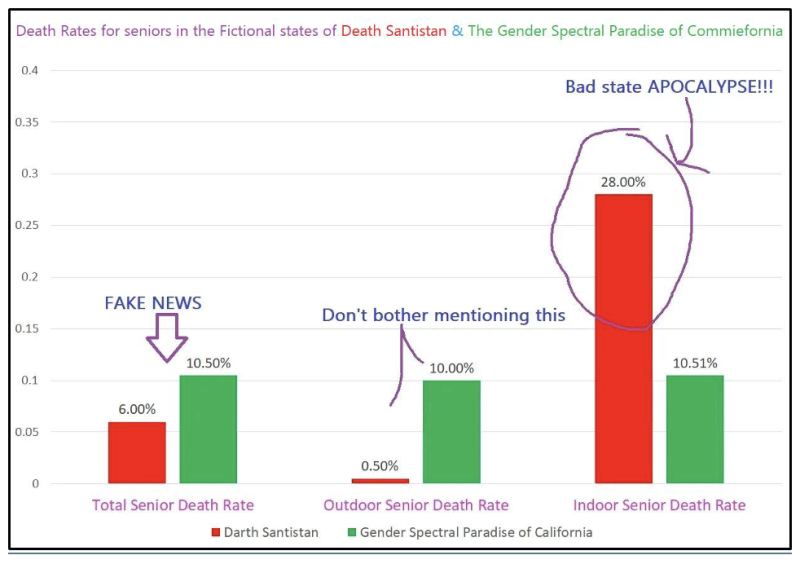
All you have to do now is to refer to the indoor senior death rate as the “population adjusted senior death rate.”
Also, you could still refer to indoor senior deaths from time to time because it’s a lot easier to propagandize with a talking point like “seniors most at risk because they are immobile were almost THREE times as likely to die in the BAD state as they were in the GOOD state.” People naturally associate seniors with being stuck indoors, so they’re unlikely to appreciate that “indoor seniors” are in reality such a small percentage of our hypothetical Death Santistan senior population.
Analysis Tactic #3: Pick optimal endpoints
Endpoints are a big deal. Officially, the primary endpoint/s of a study is/are the central finding that determines whether the study is deemed a success or a failure. An endpoint is basically a thing or metric that you’re using to assess the success/failure or the impact of whatever it is you’re studying. For example, if you’re testing a new drug to see if it stops the Dreaded Disease from killing you, the endpoint would be Dreaded Disease deaths. If the treatment group had less Dreaded Disease deaths than the control group, then the treatment works, but if they didn’t, well, that means you didn’t rig the study well enough. (That’s a bit oversimplified but you get the basic idea.)
So you gotta make sure to choose wisely when picking the endpoint/s.
Therefore, you should generally pick endpoints that have as many of the following characteristics as possible:
- Depends on subjective judgment rather than objective observation
- Naturally biased to your preferred results
- Easy to manipulate the outcome
- Easy to lie about the outcome
- Hard for people to figure out if you falsified or manipulated the outcome
- Hard to grasp/understand – especially for laypeople
For example, let’s suppose you are running a trial for the purpose of sabotaging an alternative treatment that actually works on the Dreaded Disease (which would be very bad if the regime wants a pandemic crisis to be perpetuated for a while longer). You need to show it doesn’t work. If you pick “death” as an endpoint, you could get into big trouble when the drug saves a bunch of people in the treatment group.
Instead of death, you could pick something like “time to discharge from hospital.” This endpoint fulfills all six conditions (to some degree):
- Patient dischargement is a subjective decision by the doctors (who should be on the study’s payroll), so you’re not stuck releasing patients who meet an objective standard for release.
- Dischargement is biased to your preferred results – since a higher percentage of the control group will die, this means that a higher percentage of severe cases never get discharged so they won’t increase the average time to discharge for the rest of the control group; compared to the treatment group where instead of dying, the more severely sick patients take a few extra days to recover, which increases the average time to discharge for the treatment group.
- Dischargement is very easy to manipulate – you can recruit the hospital staff involved in the study to unnecessarily delay dischargement of the treatment patients for a bit (you need to make sure that the relevant staff knows who got the treatment and therefore waits extra to discharge from the hospital).
- Time to dischargement is also fairly easy to falsify; just edit the paperwork for either date admitted to hospital and/or date discharged (and the security footage if necessary). Death is much harder to falsify, because time of death is typically something recorded very accurately and appears on the death certificate.
- ‘Time to dischargement’ is not the most intuitive metric to a layperson.
Obviously, you can do better for most of these conditions, but this conveys the basic idea.
Analysis Tactic #4: Bury alternate endpoint metrics
This one is practically self-evident: if you use ‘time to discharge’ as the endpoint but report that there was a 50% reduction in mortality in the treatment group, well, let’s just say that will raise a lot of eyebrows.
So instead of having to face tough questions about why you chose such an absurd endpoint, and why you would claim the treatment doesn’t work if you see that the treatment significantly reduced mortality, you should ideally not report the deaths anywhere in the study.
If you can’t avoid reporting the mortality stats, at least you should bury them in the middle of a random table of an appendix in a format that is very difficult to make sense of. Or better yet, sprinkle them throughout multiple data tables instead of all in one place where it is easily identified by some annoying random nerd in his basement.
Analysis Tactic #5: Employ the optimal types of analysis to get your desired results
There are as many ways to analyze data as there are gender identities or pronoun combinations. Unfortunately, an in-depth explanation for various methods cannot be distilled into a format appropriate for an Idiot’s Guide like this. Just look at some of these names:
- Balanced Design Analysis of Variance
- Beta Distribution Fitting
- Box-Cox Transformation for Two or More Groups (T-Test and One-Way ANOVA)
- Clustered Heat Maps (Double Dendrograms)
- Distribution (Weibull) Fitting
- Fuzzy Clustering
- Gamma Distribution Fitting
- General Linear Models (GLM)
- Grubbs’ Outlier Test
- Hierarchical Clustering/Dendrograms
- K-Means Clustering
- Medoid Partitioning
- Multivariate Analysis of Variance (MANOVA)
- Nondetects-Data Group Comparison
- One-Way Analysis of Covariance (ANCOVA)
- Regression Clustering
Point is, different methods of statistical analysis will yield different results. If they didn’t give different results, then there wouldn’t be so many methods. It’s all a matter of perspective. So you gotta hire yourself competent statistical gurus who know this stuff (and are loyal to the regime) for two reasons:
- You get the benefit of their expertise (which you need; remember your expertise is propagandizing, not fancy statistical analysis. A little practical humility recognizing your own limitations is crucial to being a successful propagandist; overconfidence has been the undoing of many a loyal regime lackey [and often also precipitated a lengthy vacation in an underwhelming Gulag]).
- Regime heretics cannot point to the lack of credible expertise of your statistical analysts to besmirch and impeach the credibility of regime studies. The case of Neil Ferguson stands as a cautionary tale – although he initially succeeded convincing governments around the world with his fabulous model predicting apocalyptic carnage from Covid, his utter lack of any subject matter expertise plus his lengthy history of completely delusional pandemic predictions gave the opposition firm basis to discard his models and all subsequent models pushed by various governments. They were also able to proselytize to great effect on the back of this debacle.
Analysis Tactic #6: Remove problematic data that cannot be analyzed, adjusted, or otherwise hidden
This is the same concept as kicking out subjects from a study if they are inconsistent with the regime’s mandated results; just here you’re removing the data already generated instead of the study subjects themselves. The objective is the same though: to prevent the data that doesn’t fit with what you want the study results to show from getting into the official record of the study in the first place.
IV-4. Study Rigging Option #4: Recruiting Media to Spin the Results
Regardless of what the results are, you should have ready-to-go talking points for sympathetic media outlets to go to bat for you. It doesn’t make a difference how false, misleading, etc. they are – the whole point of propaganda is to gaslight and mislead – the media simply by flooding the ecosphere with your information is a powerful force that will at minimum make it very difficult for most people to be able to unwind the lies and deceptions you are propagating rapidly throughout society.
You should be especially prepared to viciously target any scientist or academic with heretical leanings who might question anything you say, or worse, call attention to deficiencies in your study. With maximum prejudice.
Section V – Doctoring the Datasets
The other major source of Science besides for studies are the datasets and other sources of information used to make Scientific pronouncements. Data – especially official State data – is usable without a formal study bequeathing its blessing, so you must ensure that the available data, and especially the datasets that are the basis for conventional metrics commonly quoted in society by academics and laypeople alike, are in your firm control to doctor, alter and modify at will.
The following are the types of tactics you should employ to maximize the control and utility of available datasets:
V-1. Statistical ‘Fishing’
Statistical fishing is easier to just give an illustration than explain it in the abstract:
Suppose a Big Pharma company comes out with a new drug that (they claim) makes kids smarter and boosts their academic performance. Unfortunately, even though it was approved by the FDA, they know it doesn’t work, and people are beginning to suspect that there might be something fishy going on (and they’ve got billions of dollars on the line). So they come to you and offer you a hefty 7-figure paycheck to ‘prove’ that their new drug works. So you, being an audacious scientist-for-hire without any scruples (except loyalty to the regime of course), accept their offer. How do you ‘prove’ their drug works? Simple. You get the data from all the school districts in the country that shows the academic scores and the percentage of kids who took the new Pharma drug. Here’s where the ‘fishing’ part comes in: You have to look through every district until you find one or two where the academic scores are above average and more kids in that district were taking the new drug than the average (like fishing where you keep at it until you hook a fish). Then you publish your ‘study:’ “We found a correlation in District “X” where a higher percentage of kids taking the new drug led to higher academic scores.” This is baloney because every other district shows that the drug had no effect on academic scores at all, but you are neatly avoiding that by highlighting the one district where there is a correlation by random chance. (With a large enough sample size, you’re pretty much guaranteed to find one district at random where by coincidence lots of kids took the drug and the academic scores went up.)
The main lesson is that sometimes all you need is a little persistence. If you have a big dataset of many countries for instance, just go through one at a time until you discover the correlation you’re looking for. Alternatively, you can attempt a more advanced version of this tactic known as ‘P-Hacking.’
A great example of this tactic is the following CDC “study” where they went through all 50 states looking for one where they could finesse the data to show that the Covid vaccines reduced the risk of reinfection in people who already had Covid before getting the vaccine. And whaddya know, they found one (out of 50 plus a few non-state jurisdictions like Washington, DC) where they could make the data say what they wanted it to say:
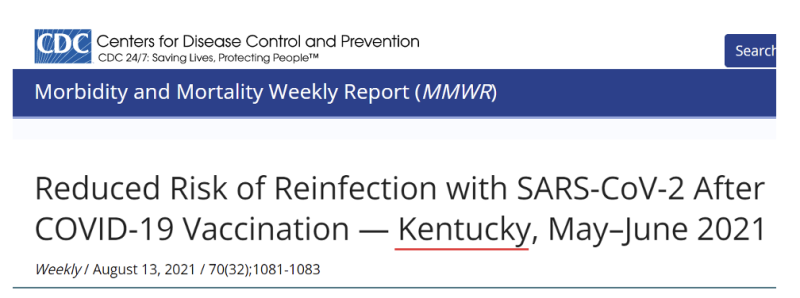
See, if the CDC was able to use more than one state to show that the Covid vaccines reduced the risk of reinfection, they would’ve (duh). But they tried and tried until they found a state that they could torture the data to show this.
By the way, there is another important lesson for propagandists here: the value of persistence. Don’t just give up if you can’t find a dataset that’s easily doctored or manipulated to bolster a regime talking point. Sometimes you gotta get creative and keep at it until you strike paydirt.
V-2. Adjust Problematic Data
Yup, we mentioned this earlier in the section about rigging studies.
If the raw data doesn’t conform to your preferred narrative, then simply ‘adjust’ it until it fits, the same way you would for a study’s internal data. Data adjusting is a routine part of science, and since very few people actually understand how it works, you can take advantage and abuse this practice.
Some fellow even published a Scientific article about the topic (it makes for interesting reading if you’re a geeky nerd):
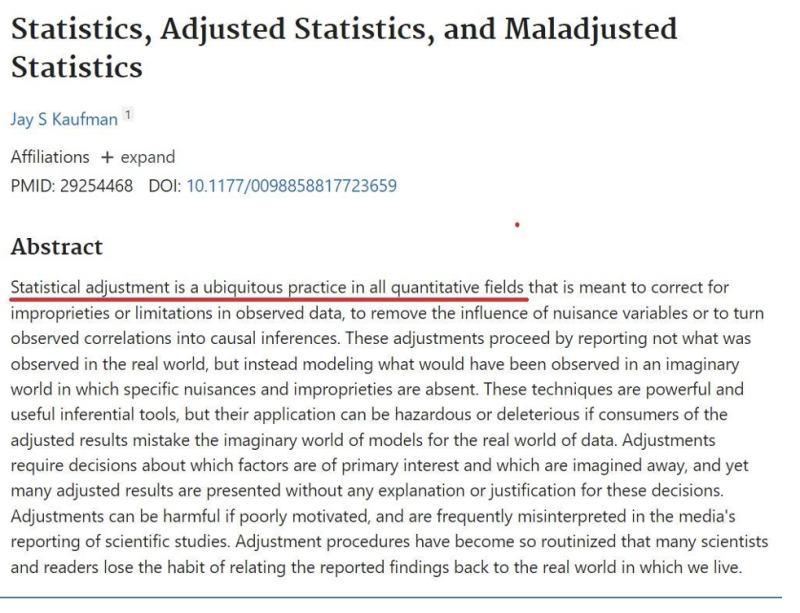
A brilliant application of this concept relates to the Global Warming Scientific establishment consensus that used to be the Global Cooling Scientific establishment consensus. How do you think that the same data which showed in 1974 that the world was heading for an irreversible Ice Age that threatened humanity’s survival now shows that there was really a *warming* trend from the exact same data that is threatening humanity’s survival??
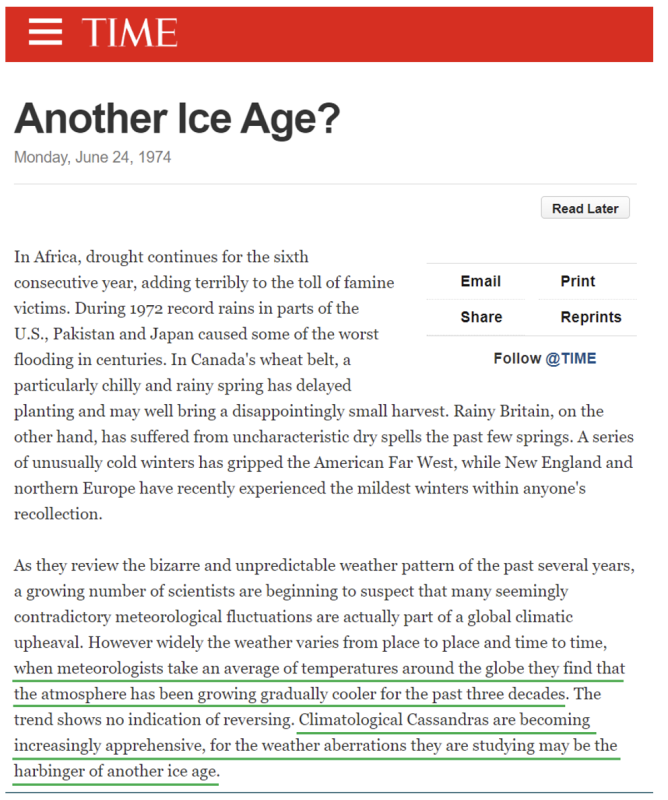
They simply ‘adjusted’ the data to make the earlier decades colder and the later decades warmer, and voila, problem solved! It’s devilishly cunning, and highly effective – observe in the chart below (from a noted regime dissident heretic) the two lines that track the average annual temperature, blue line = the raw data, the orange line = the data after the regime scientists ‘adjusted’ it:
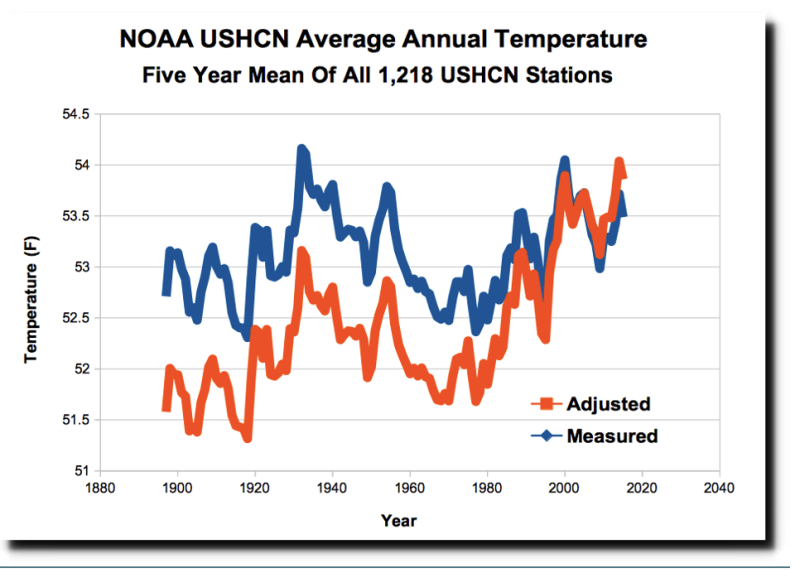
If you look at the blue line, there’s no overall warming over the past 100 years – which is very bad for the official narrative of CATASTROPHIC GLOBAL WARMING!!! However, the orange line shows a clear warming trend over the past 100 years – which is exactly the narrative.
Of course, if in the future for whatever reason it becomes pragmatic to revert back to Global Cooling, then the regime scientists over at NOAA will simply ‘readjust’ the data to make the past 100 years look like a steady cooling trend.
Point is, it’s all in the adjustments.
(Note: It’s useful to allow a few random low-profile regime science heretics to hang around because they produce data and analysis that is actually quite helpful for the regime’s own internal use, as long as you make sure they don’t start gaining prominence – then you cart them off to Guantanamo Bay without delay.)
V-3. Exclude from Official Analyses of Official Data Anything That Doesn’t Fit with Your Desired Results
Carefully vetting what gets included in your analysis is literally 101 stuff. If information or actual results threaten to undermine your preferred results, just exclude them from official analyses of the official data. So if there’s a government database that shows that, after the Glorious Vaccine, the incidence of a bunch of medical conditions went up a lot, just ignore it.
Take the VAERS (Vaccine Adverse Event Reporting System) database jointly managed by the CDC and FDA:
The CDC (pretends to) encourage reporting to VAERS medical conditions that manifest after someone gets vaccinated, “even if you are not sure that the vaccine caused the illness:”
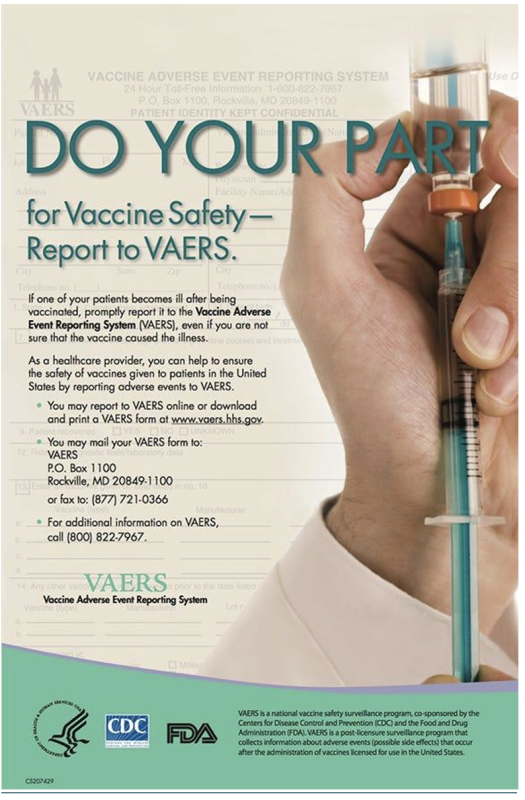
After the Covid vaccines were rolled out mid-December 2020, the VAERS entries for deaths look like this (chart shows the total # of reported deaths for all vaccines each year):
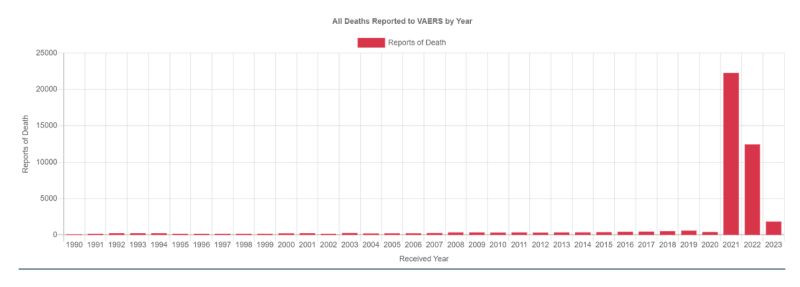
This graphic shows stats for VAERS reports of injuries/deaths from the Covid vaccines:
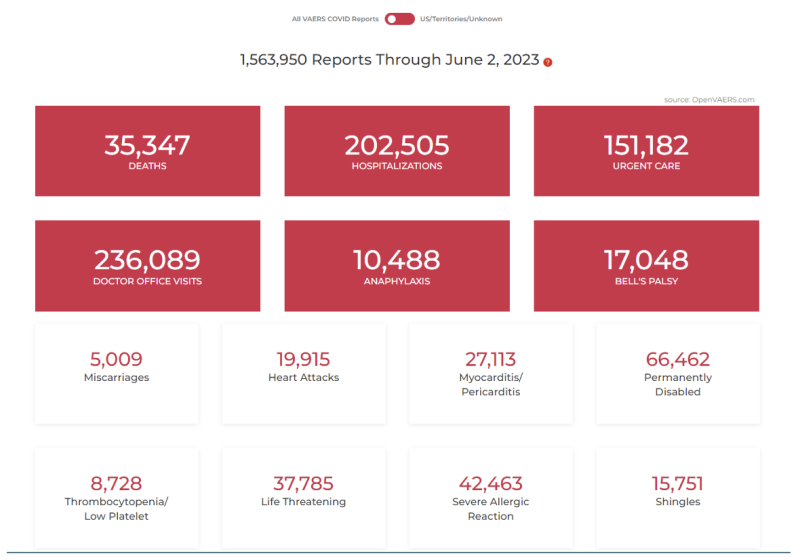
Yet, when was the last time you heard about VAERS from the CDC in any statement or analysis concerning the precious Covid vaccines?
Exactly!! The CDC (and everyone else) simply ignores VAERS (except when they from time to time issue ‘fact-checking’ pieces to debunk VAERS).
Also, make sure to relentlessly hound into oblivion anyone who dares to try and use such data to undermine the credibility of your regime analyses and proclamations. This is often a problem because inevitably there will be a bunch of people who have access to the raw data once it exists.
V-4. Piggyback on Prior Established Relationships and Differences
An easy way to jury-rig a study is to compare 2 entities that you know already have a particular difference or correlation. You can then pretend to ‘discover’ this difference or correlation but attribute it to a new factor.
So if for instance since poor states compared to rich states tend to have worse health outcomes, if the poor states happen to be less compliant with regime guidance, you can point to their worse health outcomes and blame it on them not taking the Glorious Vaccine. The media really excels at amplifying this message in particular, because they love nothing more than attributing bad outcomes to political affiliation with the “bad” political party/parties.
V-5. Control Critical Datasets Used for Scientific Research
He who controls the data controls The Science. Take care to have ironclad control over the most prominent and widely used datasets, and you will save yourself much stress and headaches. For instance, the military controls their internal datasets, and can manipulate them at will. Like DMED – they doctored this dataset all right to the point of rendering the whole thing useless. Take a look below at the following two charts showing the *SAME* DMED data for “rates of ambulatory doctor visits” for the years 2015-2018 – the left chart is the version published in 2019, the right chart shows the 2021 version – and somehow, they are not the same (red circled areas).
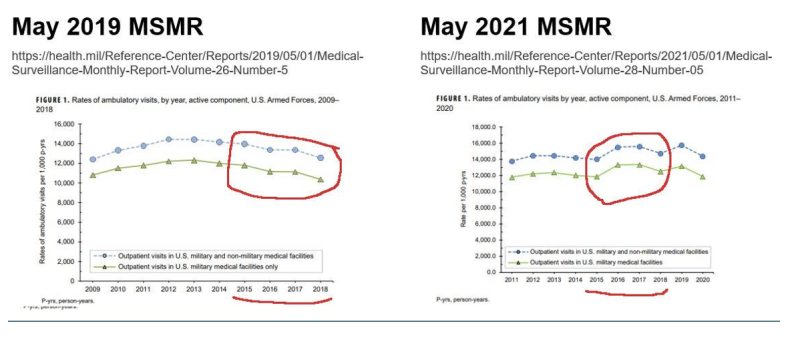
Notice the change in the 2016-2018 numbers (which you can see by the shape of the trend line)? How did the number of doctor visits that occurred in 2016 increase between 2019 and 2021????
Because the regime simply rewrote the data. That’s what you can do when you have complete control over the dataset.
It goes without saying that under no circumstance should you allow any heathen scientists access to the sacred texts or data of Science under your control – remember, you must be always vigilant lest a rogue heretical researcher perform an analysis that could invalidate or contradict The Science. The CDC leads by example here:
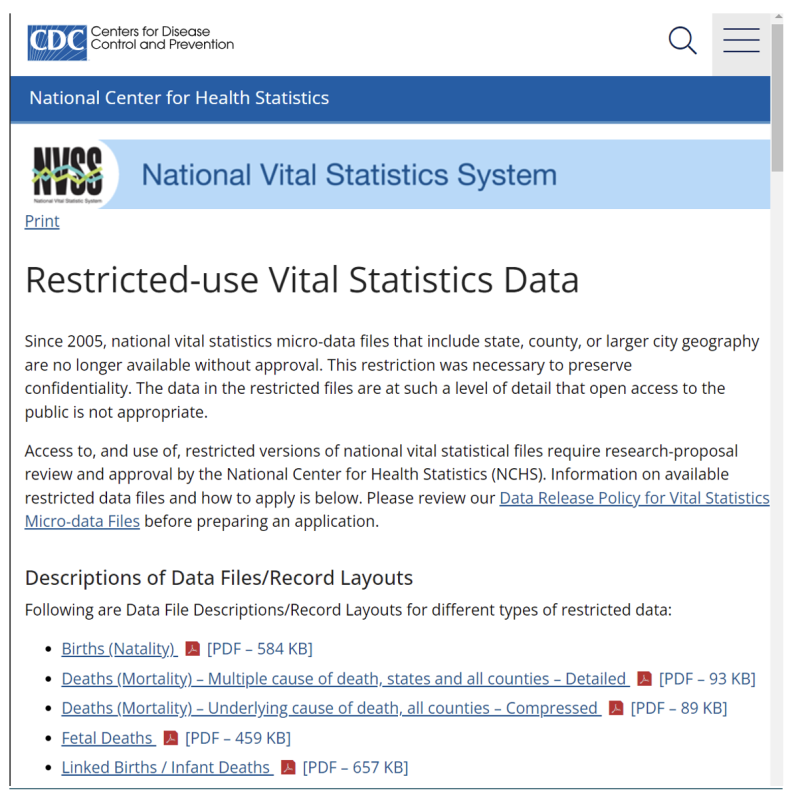
If you don’t give annoying pesky independent scientists access to the data, you don’t have to worry about them discovering things in the data that will undermine the regime narrative big-time.
Section VI – Control the Standards of Evidence
Think of yourself as a judge presiding over a criminal trial, who rules on what evidence is admissible in court, and thus can ensure that incriminating or exculpatory evidence never reaches the jury. Same idea here – by controlling the standards of evidence, you can indirectly eliminate a lot of the challenging science that’s out there without having to directly challenge or the specific claims or evidence.
VI-1. Make the Highest Quality Type of Evidence Impossible for Anyone but Regime-Approved Actors to Fulfill
This is a simple rule: Make it as difficult as humanly possible for independent scientists or researchers to conduct the type of studies deemed ‘high-quality.’
You could make it too expensive for contrarian-minded dissidents to conduct Unapproved or Heretical Science. One of the greatest coups in propaganda history was the ascendance of Random Controlled Trials as the “Gold Standard” for evidence. These typically cost many millions to conduct, foreclosing the possibility of anyone but gigantic pharmaceutical corporations (who are loyal regime actors) from running such scientific enterprises.
You can also pass laws or use government agencies to forbid unapproved high-quality studies from being conducted should a group somehow manage to secure sufficient funds to conduct such a study.
VI-2. Designate the Type of Studies Unapproved Scientists Can Conduct as “Low-Quality”
Conversely, make sure that any non-conforming science or research that can still be conducted is designated as low-quality evidence. This is usually a better alternative than banning all unapproved research outright, which will naturally make the populace suspicious of the regime and prone to accepting all kinds of wild nonsensical conspiracy theories. Rather, let them do their research, but explain that it is meaningless because it doesn’t conform to proper rules of evidence-based Science.
VI-3. Do not Articulate a Clear Standard of Evidence That Ordinary People Can Apply on Their Own
You’re going to inevitably face situations where you need the leeway to employ a double standard of evidence. If you articulate a clear and easily understandable standard, then you are neutering your own ability to doctor the science, since people can then hold you to your own professed standard. Also, as pointed out earlier, you want to condition people that the real standard is simply whatever the regime declares to be high-quality evidence rather than any remotely objective criteria.
VI-4. Persecute Insolent or Disloyal Scientists
Once in a while, a situation will arise where you may find yourself unable to impeach the credibility of research on the basis that it is poor-quality evidence. In such cases, you should instead persecute the offending scientist/s responsible for disseminating the heretical Science, thereby ceasing the dissemination and further conducting of the problematic research. This can be as innocuous as deplatforming them from social media, or as encompassing as also sending them to a Gulag never to be seen or heard from again. However you or the regime ultimately decide to remove them from the public sphere, you must make sure to also relentlessly attack their reputations and expertise (even after the seditious traitor has been eliminated). This is also a good tactic to deploy against a charismatic scientist/s who threatens the regime because he or she captures the hearts of the masses. This is true even if they seem loyal, unless you know for an absolute fact that they will never ever defect to the other side (like if you have blackmail information, or they are the heart and soul of the regime’s narrative and are fanatically committed, like the Sainted Dr Fauci). Thus you should maintain a robust spying apparatus to keep track of all the loyal regime scientists.
Section VII – The Ecclesiastical Authorities of Science
The first thing you must realize is that the concept of authority within the realm of Science is ecclesiastical in nature. The bulk of scientific discourse in society today consists of arguments from authority. So instead of resisting this, embrace it and wield it, for it is the mightiest of all weapons in the fight to control Science itself. You are the Church of Science. The regime is its Vatican. Flex your muscles and impose your will!!
You must enshrine conventions that will naturally select for only regime loyalists to ascend to positions of Scientific authority in society. This is primarily accomplished via the following methods:
VII-1. Experts Must Be Credentialed
Credentials are the first screen that weeds out most potential miscreants. By requiring credentials – which of course one can only obtain through the intermediary of the regime itself, or an institution accredited by and loyal to the regime. You must reinforce the convictions that non-credentialed experts are uniquely dangerous and ignorant, for the populace is burdened by the constant urge to seek a second opinion to regime positions and pronouncements.
VII-2. Experts Must Be Affiliated with an Institution or Organization in Good Standing
Another obvious rule. This is a good way to further weed out any potential Manchurian scientists who made it through the credentialing process.
VII-3. Experts Must Be Considered in the “Mainstream”
Rigorously enforce this social convention, for it is a potent safety net in the event that an expert breaks ranks and decides to buck the regime. Such people cannot be easily decredentialed, and sometimes it can be difficult or impractical to terminate all affiliations they might have with organizations in good standing. Thus the need for a disqualification that is not dependent on either of those. Declaring him outside the mainstream is a fairly potent way of defoliating such an expert of his authority.
VII-4. Enforce Scientific Consensus
Another powerful way to control who wields scientific authority is to enforce compliance to a concocted “consensus,” branding anyone who deviates from said consensus as an unmitigated, incorrigible heretic of the most deviant sort. It’s an external tool that can be extremely useful to dethrone wayward credentialed scientists. “Consensus” rings powerfully in the ears and hearts of laypeople, and provides them easy justification to not raise questions if the regime decides to suddenly defrock a previously highly-esteemed Scientist.
Afterword
The art of propaganda is a broad subject encompassing multiple disciplines. Do not expect that you will master it overnight. Expect that you will make mistakes – that’s how you learn what works (and therefore also make sure to always have someone else whom you can pin the blame for your mistakes on).
Luckily for you, the vast majority of the citizens are intellectual sheep. This principle was brilliantly demonstrated by Obamacare’s chief architect Professor Jonathan Gruber.
However, Professor Gruber had a penchant for explaining too much, and too clearly, in recorded speeches. Of course, there’s nothing wrong with explaining controversial matters in clear language to young regime students that are key to understanding how regime politics function, because they’ll need to have a firm grasp of these things if they’re to be productive regime workers. It becomes an issue however when these speeches are recorded on video that is accessible to the general public who you are supposed to be duping:
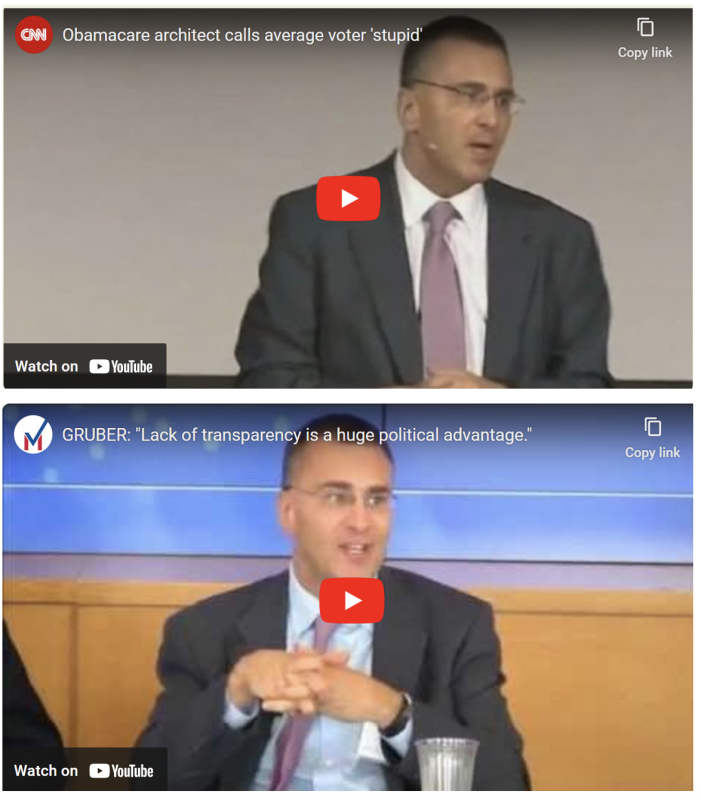
You would think that after the guy who wrote a deeply unpopular law (at the time) was caught on numerous videos boasting about how it was a “clever exploitation of the lack of economic understanding of the American voter” and how “the stupidity of the American voter” was critical to being able to pass off a tax increase as not a tax increase (which are both 100% true as stated earlier), that the politicians would be forced to pull the law and try again in a few years after the hullabaloo had died down.
Except that, as it turns out, you can usually count on not only the unmitigated stupidity of the average voter, but also on their lack of any short-term memory, their lack of a sense of self-preservation, and their commitment to political ideology above all. Did Obamacare get undone, or even delayed? Nope. So even if you get busted big time, you will probably be okay. (Especially if you have cultivated a compliant mainstream media that serves the regime loyally.)
You can also take comfort that propaganda is naturally a self-correcting enterprise – when mistakes are made, you simply unleash more propaganda and gaslighting to cover up or otherwise mitigate those mistakes. Observe how the regime officials shifted from exalting Professor Gruber to claiming he was wholly insignificant, without batting an eyelash, and without the faintest hint of embarrassment at the rank naked hypocrisy of their irreconcilable positions:
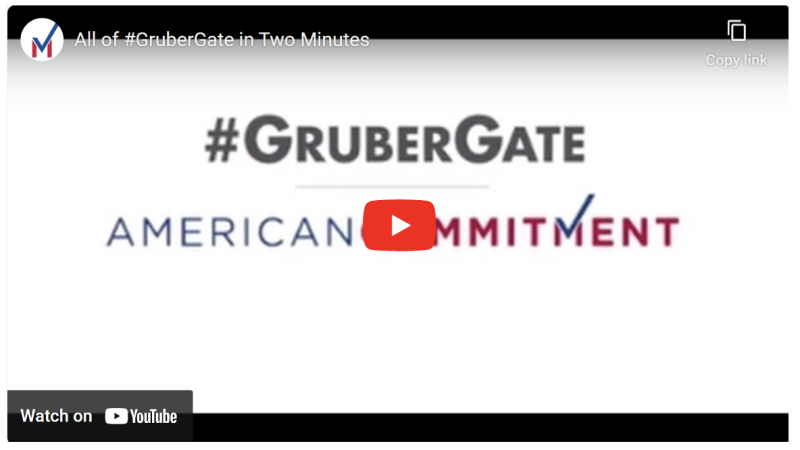
(You should take care not to be too cavalier about making errors though, or you may find yourself relocated to a Soviet Gulag or CIA Black Ops site in Morocco.)
Together, we can make the world a better place for those destined to be a part of the newly reset humanity.
Published under a Creative Commons Attribution 4.0 International License
For reprints, please set the canonical link back to the original Brownstone Institute Article and Author.